158 lines
7.3 KiB
Markdown
158 lines
7.3 KiB
Markdown
<!--Copyright 2023 The HuggingFace Team. All rights reserved.
|
|
|
|
Licensed under the Apache License, Version 2.0 (the "License"); you may not use this file except in compliance with
|
|
the License. You may obtain a copy of the License at
|
|
|
|
http://www.apache.org/licenses/LICENSE-2.0
|
|
|
|
Unless required by applicable law or agreed to in writing, software distributed under the License is distributed on
|
|
an "AS IS" BASIS, WITHOUT WARRANTIES OR CONDITIONS OF ANY KIND, either express or implied. See the License for the
|
|
specific language governing permissions and limitations under the License.
|
|
|
|
⚠️ Note that this file is in Markdown but contain specific syntax for our doc-builder (similar to MDX) that may not be
|
|
rendered properly in your Markdown viewer.
|
|
|
|
-->
|
|
|
|
# SAM
|
|
|
|
## Overview
|
|
|
|
SAM (Segment Anything Model) was proposed in [Segment Anything](https://arxiv.org/pdf/2304.02643v1.pdf) by Alexander Kirillov, Eric Mintun, Nikhila Ravi, Hanzi Mao, Chloe Rolland, Laura Gustafson, Tete Xiao, Spencer Whitehead, Alex Berg, Wan-Yen Lo, Piotr Dollar, Ross Girshick.
|
|
|
|
The model can be used to predict segmentation masks of any object of interest given an input image.
|
|
|
|
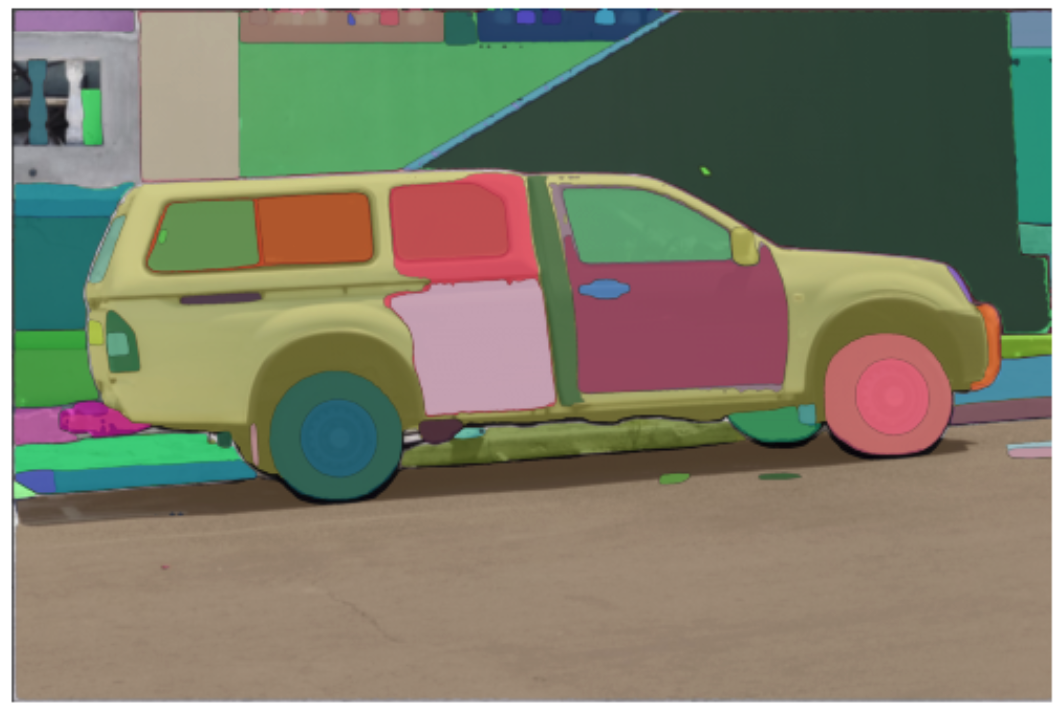
|
|
|
|
The abstract from the paper is the following:
|
|
|
|
*We introduce the Segment Anything (SA) project: a new task, model, and dataset for image segmentation. Using our efficient model in a data collection loop, we built the largest segmentation dataset to date (by far), with over 1 billion masks on 11M licensed and privacy respecting images. The model is designed and trained to be promptable, so it can transfer zero-shot to new image distributions and tasks. We evaluate its capabilities on numerous tasks and find that its zero-shot performance is impressive -- often competitive with or even superior to prior fully supervised results. We are releasing the Segment Anything Model (SAM) and corresponding dataset (SA-1B) of 1B masks and 11M images at [https://segment-anything.com](https://segment-anything.com) to foster research into foundation models for computer vision.*
|
|
|
|
Tips:
|
|
|
|
- The model predicts binary masks that states the presence or not of the object of interest given an image.
|
|
- The model predicts much better results if input 2D points and/or input bounding boxes are provided
|
|
- You can prompt multiple points for the same image, and predict a single mask.
|
|
- Fine-tuning the model is not supported yet
|
|
- According to the paper, textual input should be also supported. However, at this time of writing this seems to be not supported according to [the official repository](https://github.com/facebookresearch/segment-anything/issues/4#issuecomment-1497626844).
|
|
|
|
|
|
This model was contributed by [ybelkada](https://huggingface.co/ybelkada) and [ArthurZ](https://huggingface.co/ArthurZ).
|
|
The original code can be found [here](https://github.com/facebookresearch/segment-anything).
|
|
|
|
Below is an example on how to run mask generation given an image and a 2D point:
|
|
|
|
```python
|
|
import torch
|
|
from PIL import Image
|
|
import requests
|
|
from transformers import SamModel, SamProcessor
|
|
|
|
device = "cuda" if torch.cuda.is_available() else "cpu"
|
|
model = SamModel.from_pretrained("facebook/sam-vit-huge").to(device)
|
|
processor = SamProcessor.from_pretrained("facebook/sam-vit-huge")
|
|
|
|
img_url = "https://huggingface.co/ybelkada/segment-anything/resolve/main/assets/car.png"
|
|
raw_image = Image.open(requests.get(img_url, stream=True).raw).convert("RGB")
|
|
input_points = [[[450, 600]]] # 2D location of a window in the image
|
|
|
|
inputs = processor(raw_image, input_points=input_points, return_tensors="pt").to(device)
|
|
with torch.no_grad():
|
|
outputs = model(**inputs)
|
|
|
|
masks = processor.image_processor.post_process_masks(
|
|
outputs.pred_masks.cpu(), inputs["original_sizes"].cpu(), inputs["reshaped_input_sizes"].cpu()
|
|
)
|
|
scores = outputs.iou_scores
|
|
```
|
|
|
|
You can also process your own masks alongside the input images in the processor to be passed to the model.
|
|
|
|
```python
|
|
import torch
|
|
from PIL import Image
|
|
import requests
|
|
from transformers import SamModel, SamProcessor
|
|
|
|
device = "cuda" if torch.cuda.is_available() else "cpu"
|
|
model = SamModel.from_pretrained("facebook/sam-vit-huge").to(device)
|
|
processor = SamProcessor.from_pretrained("facebook/sam-vit-huge")
|
|
|
|
img_url = "https://huggingface.co/ybelkada/segment-anything/resolve/main/assets/car.png"
|
|
raw_image = Image.open(requests.get(img_url, stream=True).raw).convert("RGB")
|
|
mask_url = "https://huggingface.co/ybelkada/segment-anything/resolve/main/assets/car.png"
|
|
segmentation_map = Image.open(requests.get(mask_url, stream=True).raw).convert("RGB")
|
|
input_points = [[[450, 600]]] # 2D location of a window in the image
|
|
|
|
inputs = processor(raw_image, input_points=input_points, segmentation_maps=mask, return_tensors="pt").to(device)
|
|
with torch.no_grad():
|
|
outputs = model(**inputs)
|
|
|
|
masks = processor.image_processor.post_process_masks(
|
|
outputs.pred_masks.cpu(), inputs["original_sizes"].cpu(), inputs["reshaped_input_sizes"].cpu()
|
|
)
|
|
scores = outputs.iou_scores
|
|
```
|
|
|
|
## Resources
|
|
|
|
A list of official Hugging Face and community (indicated by 🌎) resources to help you get started with SAM.
|
|
|
|
- [Demo notebook](https://github.com/huggingface/notebooks/blob/main/examples/segment_anything.ipynb) for using the model.
|
|
- [Demo notebook](https://github.com/huggingface/notebooks/blob/main/examples/automatic_mask_generation.ipynb) for using the automatic mask generation pipeline.
|
|
- [Demo notebook](https://github.com/NielsRogge/Transformers-Tutorials/blob/master/SAM/Run_inference_with_MedSAM_using_HuggingFace_Transformers.ipynb) for inference with MedSAM, a fine-tuned version of SAM on the medical domain. 🌎
|
|
- [Demo notebook](https://github.com/NielsRogge/Transformers-Tutorials/blob/master/SAM/Fine_tune_SAM_(segment_anything)_on_a_custom_dataset.ipynb) for fine-tuning the model on custom data. 🌎
|
|
|
|
## SlimSAM
|
|
|
|
SlimSAM, a pruned version of SAM, was proposed in [0.1% Data Makes Segment Anything Slim](https://arxiv.org/abs/2312.05284) by Zigeng Chen et al. SlimSAM reduces the size of the SAM models considerably while maintaining the same performance.
|
|
|
|
Checkpoints can be found on the [hub](https://huggingface.co/models?other=slimsam), and they can be used as a drop-in replacement of SAM.
|
|
|
|
## Grounded SAM
|
|
|
|
One can combine [Grounding DINO](grounding-dino) with SAM for text-based mask generation as introduced in [Grounded SAM: Assembling Open-World Models for Diverse Visual Tasks](https://arxiv.org/abs/2401.14159). You can refer to this [demo notebook](https://github.com/NielsRogge/Transformers-Tutorials/blob/master/Grounding%20DINO/GroundingDINO_with_Segment_Anything.ipynb) 🌍 for details.
|
|
|
|
<img src="https://huggingface.co/datasets/huggingface/documentation-images/resolve/main/transformers/model_doc/grounded_sam.png"
|
|
alt="drawing" width="900"/>
|
|
|
|
<small> Grounded SAM overview. Taken from the <a href="https://github.com/IDEA-Research/Grounded-Segment-Anything">original repository</a>. </small>
|
|
|
|
## SamConfig
|
|
|
|
[[autodoc]] SamConfig
|
|
|
|
## SamVisionConfig
|
|
|
|
[[autodoc]] SamVisionConfig
|
|
|
|
## SamMaskDecoderConfig
|
|
|
|
[[autodoc]] SamMaskDecoderConfig
|
|
|
|
## SamPromptEncoderConfig
|
|
|
|
[[autodoc]] SamPromptEncoderConfig
|
|
|
|
|
|
## SamProcessor
|
|
|
|
[[autodoc]] SamProcessor
|
|
|
|
|
|
## SamImageProcessor
|
|
|
|
[[autodoc]] SamImageProcessor
|
|
|
|
|
|
## SamModel
|
|
|
|
[[autodoc]] SamModel
|
|
- forward
|
|
|
|
|
|
## TFSamModel
|
|
|
|
[[autodoc]] TFSamModel
|
|
- call
|