4.5 KiB
Mask2Former
Overview
The Mask2Former model was proposed in Masked-attention Mask Transformer for Universal Image Segmentation by Bowen Cheng, Ishan Misra, Alexander G. Schwing, Alexander Kirillov, Rohit Girdhar. Mask2Former is a unified framework for panoptic, instance and semantic segmentation and features significant performance and efficiency improvements over MaskFormer.
The abstract from the paper is the following:
Image segmentation groups pixels with different semantics, e.g., category or instance membership. Each choice of semantics defines a task. While only the semantics of each task differ, current research focuses on designing specialized architectures for each task. We present Masked-attention Mask Transformer (Mask2Former), a new architecture capable of addressing any image segmentation task (panoptic, instance or semantic). Its key components include masked attention, which extracts localized features by constraining cross-attention within predicted mask regions. In addition to reducing the research effort by at least three times, it outperforms the best specialized architectures by a significant margin on four popular datasets. Most notably, Mask2Former sets a new state-of-the-art for panoptic segmentation (57.8 PQ on COCO), instance segmentation (50.1 AP on COCO) and semantic segmentation (57.7 mIoU on ADE20K).
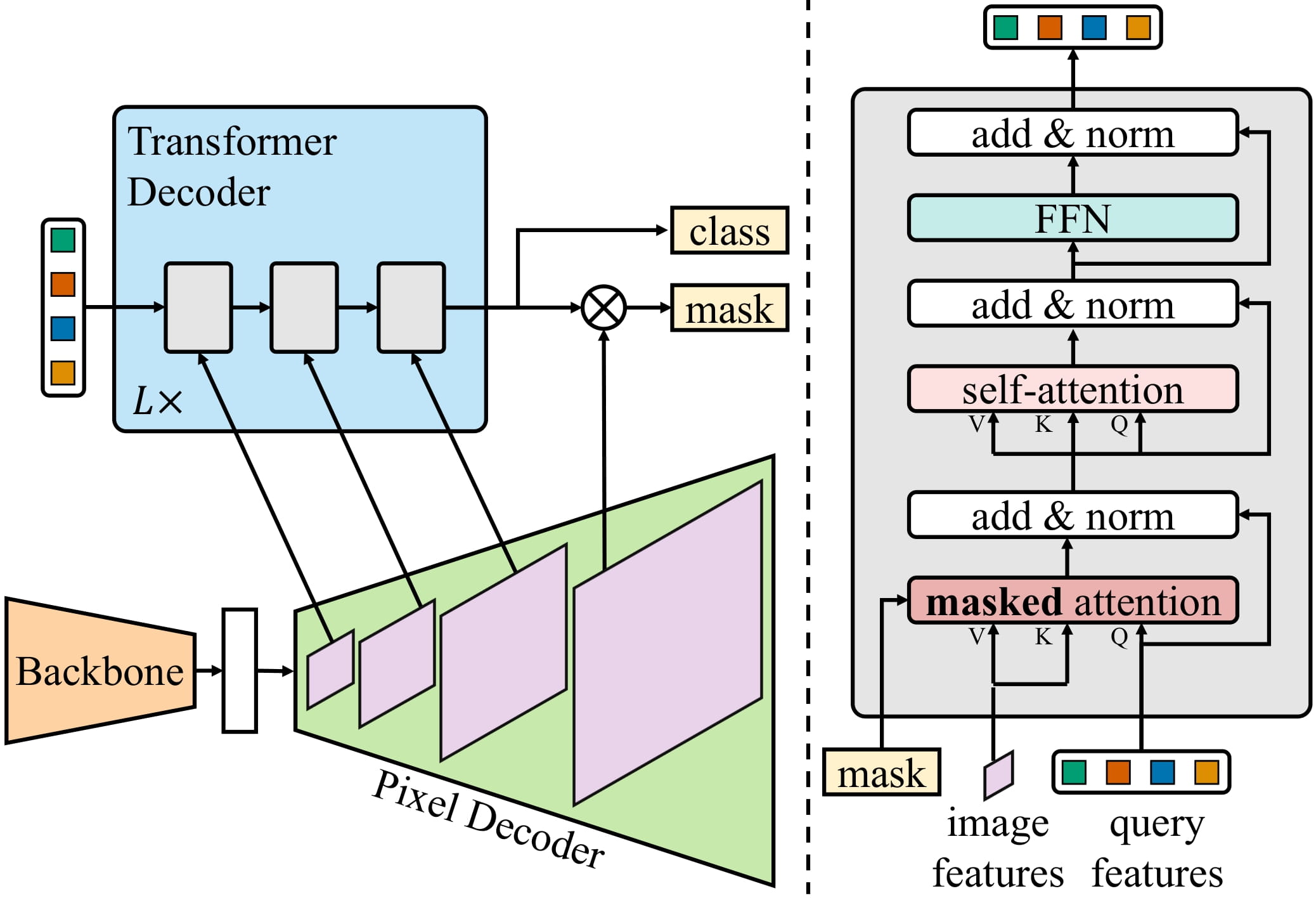
Mask2Former architecture. Taken from the original paper.
This model was contributed by Shivalika Singh and Alara Dirik. The original code can be found here.
Usage tips
- Mask2Former uses the same preprocessing and postprocessing steps as MaskFormer. Use [
Mask2FormerImageProcessor
] or [AutoImageProcessor
] to prepare images and optional targets for the model. - To get the final segmentation, depending on the task, you can call [
~Mask2FormerImageProcessor.post_process_semantic_segmentation
] or [~Mask2FormerImageProcessor.post_process_instance_segmentation
] or [~Mask2FormerImageProcessor.post_process_panoptic_segmentation
]. All three tasks can be solved using [Mask2FormerForUniversalSegmentation
] output, panoptic segmentation accepts an optionallabel_ids_to_fuse
argument to fuse instances of the target object/s (e.g. sky) together.
Resources
A list of official Hugging Face and community (indicated by 🌎) resources to help you get started with Mask2Former.
- Demo notebooks regarding inference + fine-tuning Mask2Former on custom data can be found here.
If you're interested in submitting a resource to be included here, please feel free to open a Pull Request and we will review it. The resource should ideally demonstrate something new instead of duplicating an existing resource.
Mask2FormerConfig
autodoc Mask2FormerConfig
MaskFormer specific outputs
autodoc models.mask2former.modeling_mask2former.Mask2FormerModelOutput
autodoc models.mask2former.modeling_mask2former.Mask2FormerForUniversalSegmentationOutput
Mask2FormerModel
autodoc Mask2FormerModel - forward
Mask2FormerForUniversalSegmentation
autodoc Mask2FormerForUniversalSegmentation - forward
Mask2FormerImageProcessor
autodoc Mask2FormerImageProcessor - preprocess - encode_inputs - post_process_semantic_segmentation - post_process_instance_segmentation - post_process_panoptic_segmentation