![]() |
||
---|---|---|
assets | ||
basic_demo | ||
composite_demo | ||
fewshot-data | ||
finetune_demo | ||
models/__pycache__ | ||
openai_demo | ||
utils | ||
LICENSE | ||
MODEL_LICENSE | ||
README.md | ||
README_zh.md | ||
output.png | ||
requirements.txt |
README.md
CogVLM & CogAgent
🔥🔥🔥 🆕 2023/12/15
: CogAgent Officially Launched! CogAgent is an image understanding model developed based on CogVLM. It features visual-based GUI Agent capabilities and has further enhancements in image understanding. It supports image input with a resolution of 1120*1120, and possesses multiple abilities including multi-turn dialogue with images, GUI Agent, Grounding, and more.
🌟 Jump to detailed introduction: Introduction to CogVLM, 🆕 Introduction to CogAgent
CogVLM🌐 Web Demo: this link 📖 Paper: CogVLM: Visual Expert for Pretrained Language Models CogVLM is a powerful open-source visual language model (VLM). CogVLM-17B has 10 billion visual parameters and 7 billion language parameters, supporting image understanding and multi-turn dialogue with a resolution of 490*490. CogVLM-17B achieves state-of-the-art performance on 10 classic cross-modal benchmarks, including NoCaps, Flicker30k captioning, RefCOCO, RefCOCO+, RefCOCOg, Visual7W, GQA, ScienceQA, VizWiz VQA and TDIUC. |
CogAgent🌐 Web Demo: Coming soon 📖 Paper: CogAgent: A Visual Language Model for GUI Agents CogAgent is an open-source visual language model improved based on CogVLM. CogAgent-18B has 11 billion visual parameters and 7 billion language parameters, supporting image understanding at a resolution of 1120*1120. On top of the capabilities of CogVLM, it further possesses GUI image Agent capabilities. CogAgent-18B achieves state-of-the-art generalist performance on 9 classic cross-modal benchmarks, including VQAv2, OK-VQ, TextVQA, ST-VQA, ChartQA, infoVQA, DocVQA, MM-Vet, and POPE. It significantly surpasses existing models on GUI operation datasets including AITW and Mind2Web. |
Table of Contents
- CogVLM & CogAgent
Release
-
🔥🔥🔥 News:
2023/12/15
: CogAgent Officially Launched! CogAgent is an image understanding model developed based on CogVLM. It features visual-based GUI Agent capabilities and has further enhancements in image understanding. It supports image input with a resolution of 1120*1120, and possesses multiple abilities including multi-turn dialogue with images, GUI Agent, Grounding, and more. -
🔥 News:
2023/12/8
We have updated the checkpoint of cogvlm-grounding-generalist to cogvlm-grounding-generalist-v1.1, with image augmentation during training, therefore more robust. See details. -
🔥 News:
2023/12/7
CogVLM supports 4-bit quantization now! You can inference with just 11GB GPU memory! See details. -
🔥 News:
2023/11/20
We have updated the checkpoint of cogvlm-chat to cogvlm-chat-v1.1, unified the versions of chat and VQA, and refreshed the SOTA on various datasets. See details -
News:
2023/11/20
We release cogvlm-chat, cogvlm-grounding-generalist/base, cogvlm-base-490/224 on 🤗Huggingface. you can infer with transformers in a few lines of code now! -
2023/10/27
CogVLM bilingual version is available online! Welcome to try it out! -
2023/10/5
CogVLM-17B released。
Get Started
Option 1: Inference Using Web Demo.
-
Click here to enter CogAgent Web Demo (Coming soon)
-
Click here to enter CogVLM Web Demo。
If you need to use Agent and Grounding functions, please refer to Cookbook - Task Prompts
Option 2:Deploy CogVLM / CogAgent by yourself
We support two GUIs for model inference, CLI and web demo . If you want to use it in your python code, it is easy to modify the CLI scripts for your case.
First, we need to install the dependencies.
# CUDA >= 11.8
pip install -r requirements.txt
python -m spacy download en_core_web_sm
All code for inference is located under the basic_demo/
directory. Please switch to this directory first before proceeding with further operations.
Situation 2.1 CLI (SAT version)
Run CLI demo via:
# CogAgent
python cli_demo_sat.py --from_pretrained cogagent-chat --version chat --bf16 --stream_chat
# CogVLM
python cli_demo_sat.py --from_pretrained cogvlm-chat --version chat_old --bf16 --stream_chat
python cli_demo_sat.py --from_pretrained cogvlm-grounding-generalist --version base --bf16 --stream_chat
The program will automatically download the sat model and interact in the command line. You can generate replies by entering instructions and pressing enter.
Enter clear
to clear the conversation history and stop
to stop the program.
We also support model parallel inference, which splits model to multiple (2/4/8) GPUs. --nproc-per-node=[n]
in the following command controls the number of used GPUs.
torchrun --standalone --nnodes=1 --nproc-per-node=2 cli_demo_sat.py --from_pretrained cogagent-chat --version chat --bf16
-
If you want to manually download the weights, you can replace the path after
--from_pretrained
with the model path. -
Our model supports SAT's 4-bit quantization and 8-bit quantization. You can change
--bf16
to--fp16
, or--fp16 --quant 4
, or--fp16 --quant 8
.For example
python cli_demo_sat.py --from_pretrained cogagent-chat --fp16 --quant 8 --stream_chat python cli_demo_sat.py --from_pretrained cogvlm-chat-v1.1 --fp16 --quant 4 --stream_chat # In SAT version,--quant should be used with --fp16
-
The program provides the following hyperparameters to control the generation process:
usage: cli_demo_sat.py [-h] [--max_length MAX_LENGTH] [--top_p TOP_P] [--top_k TOP_K] [--temperature TEMPERATURE] optional arguments: -h, --help show this help message and exit --max_length MAX_LENGTH max length of the total sequence --top_p TOP_P top p for nucleus sampling --top_k TOP_K top k for top k sampling --temperature TEMPERATURE temperature for sampling
-
Click here to view the correspondence between different models and the
--version
parameter.
Situation 2.2 CLI (Huggingface version)
Run CLI demo via:
# CogAgent
python cli_demo_hf.py --from_pretrained THUDM/cogagent-chat-hf --bf16
# CogVLM
python cli_demo_hf.py --from_pretrained THUDM/cogvlm-chat-hf --bf16
python cli_demo_hf.py --from_pretrained THUDM/cogvlm-grounding-generalist --bf16
-
If you want to manually download the weights, you can replace the path after
--from_pretrained
with the model path. -
You can change
--bf16
to--fp16
, or--quant 4
. For example, our model supports Huggingface's 4-bit quantization:python cli_demo_hf.py --from_pretrained THUDM/cogvlm-chat-hf --quant 4
Situation 2.3 Web Demo
We also offer a local web demo based on Gradio. First, install Gradio by running: pip install gradio
. Then download and enter this repository and run web_demo.py
. See the next section for detailed usage:
python web_demo.py --from_pretrained cogagent-chat --version chat --bf16
python web_demo.py --from_pretrained cogvlm-chat-v1.1 --version chat_old --bf16
python web_demo.py --from_pretrained cogvlm-grounding-generalist --version base --bf16
The GUI of the web demo looks like:
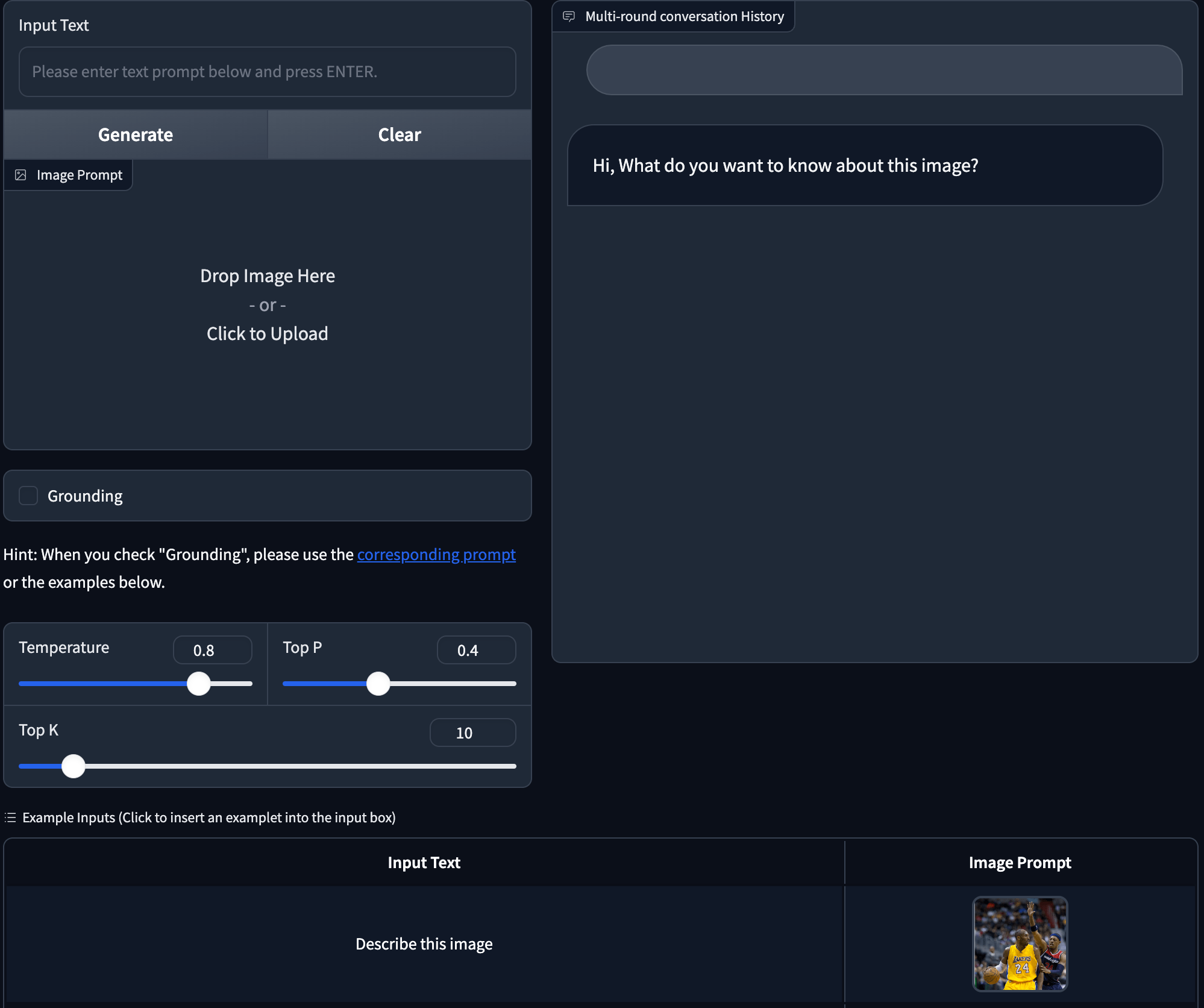
Option 3:Finetuning CogAgent / CogVLM
You may want to use CogVLM in your own task, which needs a different output style or domain knowledge. All code for finetuning is located under the finetune_demo/
directory.
We here provide a finetuning example for Captcha Recognition using lora.
-
Start by downloading the Captcha Images dataset. Once downloaded, extract the contents of the ZIP file.
-
To create a train/validation/test split in the ratio of 80/5/15, execute the following:
python utils/split_dataset.py
-
Start the fine-tuning process with this command:
bash finetune_demo/finetune_(cogagent/cogvlm)_lora.sh
-
Merge the model to
model_parallel_size=1
: (replace the 4 below with your trainingMP_SIZE
)torchrun --standalone --nnodes=1 --nproc-per-node=4 utils/merge_model.py --version base --bf16 --from_pretrained ./checkpoints/merged_lora_(cogagent/cogvlm490/cogvlm224)
-
Evaluate the performance of your model.
bash finetune_demo/evaluate_(cogagent/cogvlm).sh
Option 4: OpenAI Vision format
We provide the same API examples as GPT-4V
, which you can view in openai_demo
.
- First, start the node
python openai_demo/openai_api.py
- Next, run the request example node, which is an example of a continuous dialogue
python openai_demo/openai_api_request.py
- You will get output similar to the following
This image showcases a tranquil natural scene with a wooden pathway leading through a field of lush green grass. In the distance, there are trees and some scattered structures, possibly houses or small buildings. The sky is clear with a few scattered clouds, suggesting a bright and sunny day.
Hardware requirement
-
Model Inference:
For INT4 quantization: 1 * RTX 3090(24G) (CogAgent takes ~ 12.6GB, CogVLM takes ~ 11GB)
For FP16: 1 * A100(80G) or 2 * RTX 3090(24G)
-
Finetuning:
For FP16: 4 * A100(80G) [Recommend] or 8* RTX 3090(24G).
Model checkpoints
If you run the basic_demo/cli_demo*.py
from the code repository, it will automatically download SAT or Hugging Face weights. Alternatively, you can choose to manually download the necessary weights.
-
CogAgent
Model name Input resolution Introduction Huggingface model SAT model cogagent-chat 1120 Chat version of CogAgent. Supports GUI Agent, multiple-round chat and visual grounding. link link -
CogVLM
Model name Input resolution Introduction Huggingface model SAT model cogvlm-chat-v1.1 490 Supports multiple rounds of chat and vqa simultaneously, with different prompts. link link cogvlm-base-224 224 The original checkpoint after text-image pretraining. link link cogvlm-base-490 490 Amplify the resolution to 490 through position encoding interpolation from cogvlm-base-224
.link link cogvlm-grounding-generalist 490 This checkpoint supports different visual grounding tasks, e.g. REC, Grounding Captioning, etc. link link
Introduction to CogVLM
-
CogVLM is a powerful open-source visual language model (VLM). CogVLM-17B has 10 billion vision parameters and 7 billion language parameters.
-
CogVLM-17B achieves state-of-the-art performance on 10 classic cross-modal benchmarks, including NoCaps, Flicker30k captioning, RefCOCO, RefCOCO+, RefCOCOg, Visual7W, GQA, ScienceQA, VizWiz VQA and TDIUC, and rank the 2nd on VQAv2, OKVQA, TextVQA, COCO captioning, etc., surpassing or matching PaLI-X 55B. CogVLM can also chat with you about images.
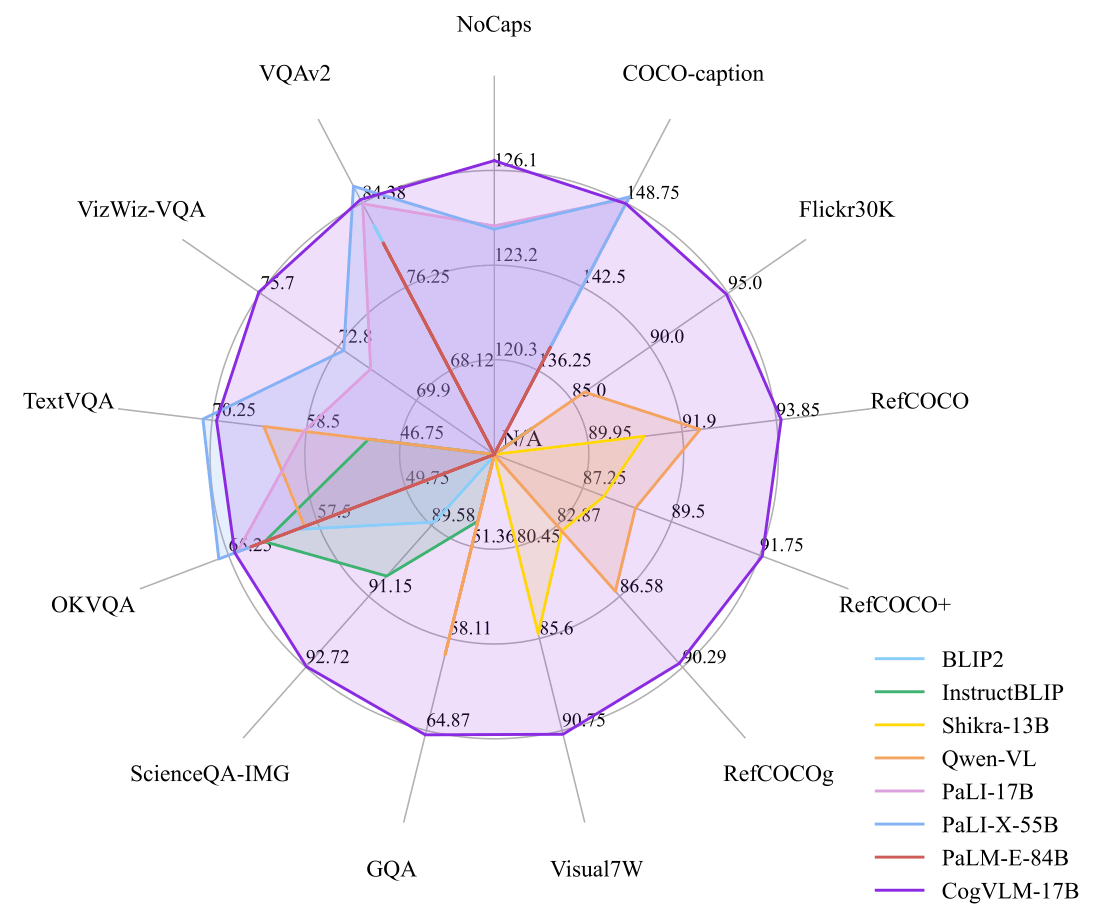
Click to view results on MM-VET, POPE, TouchStone.
Method | LLM | MM-VET | POPE(adversarial) | TouchStone |
BLIP-2 | Vicuna-13B | 22.4 | - | - |
Otter | MPT-7B | 24.7 | - | - |
MiniGPT4 | Vicuna-13B | 24.4 | 70.4 | 531.7 |
InstructBLIP | Vicuna-13B | 25.6 | 77.3 | 552.4 |
LLaMA-Adapter v2 | LLaMA-7B | 31.4 | - | 590.1 |
LLaVA | LLaMA2-7B | 28.1 | 66.3 | 602.7 |
mPLUG-Owl | LLaMA-7B | - | 66.8 | 605.4 |
LLaVA-1.5 | Vicuna-13B | 36.3 | 84.5 | - |
Emu | LLaMA-13B | 36.3 | - | - |
Qwen-VL-Chat | - | - | - | 645.2 |
DreamLLM | Vicuna-7B | 35.9 | 76.5 | - |
CogVLM | Vicuna-7B | 52.8 | 87.6 | 742.0 |
Click to view results of cogvlm-grounding-generalist-v1.1.
RefCOCO | RefCOCO+ | RefCOCOg | Visual7W | ||||||
val | testA | testB | val | testA | testB | val | test | test | |
cogvim-grounding-generalist | 92.51 | 93.95 | 88.73 | 87.52 | 91.81 | 81.43 | 89.46 | 90.09 | 90.96 |
cogvim-grounding-generalist-v1.1 | **92.76** | **94.75** | **88.99** | **88.68** | **92.91** | **83.39** | **89.75** | **90.79** | **91.05** |
Examples
-
CogVLM can accurately describe images in details with very few hallucinations.
Click for comparison with LLAVA-1.5 and MiniGPT-4.
-
CogVLM can understand and answer various types of questions, and has a visual grounding version.

- CogVLM sometimes captures more detailed content than GPT-4V(ision).

Introduction to CogAgent
CogAgent is an open-source visual language model improved based on CogVLM. CogAgent-18B has 11 billion visual parameters and 7 billion language parameters
CogAgent-18B achieves state-of-the-art generalist performance on 9 classic cross-modal benchmarks, including VQAv2, OK-VQ, TextVQA, ST-VQA, ChartQA, infoVQA, DocVQA, MM-Vet, and POPE. It significantly surpasses existing models on GUI operation datasets such as AITW and Mind2Web.
In addition to all the features already present in CogVLM (visual multi-round dialogue, visual grounding), CogAgent:
-
Supports higher resolution visual input and dialogue question-answering. It supports ultra-high-resolution image inputs of 1120x1120.
-
Possesses the capabilities of a visual Agent, being able to return a plan, next action, and specific operations with coordinates for any given task on any GUI screenshot.
-
Enhanced GUI-related question-answering capabilities, allowing it to handle questions about any GUI screenshot, such as web pages, PC apps, mobile applications, etc.
-
Enhanced capabilities in OCR-related tasks through improved pre-training and fine-tuning.
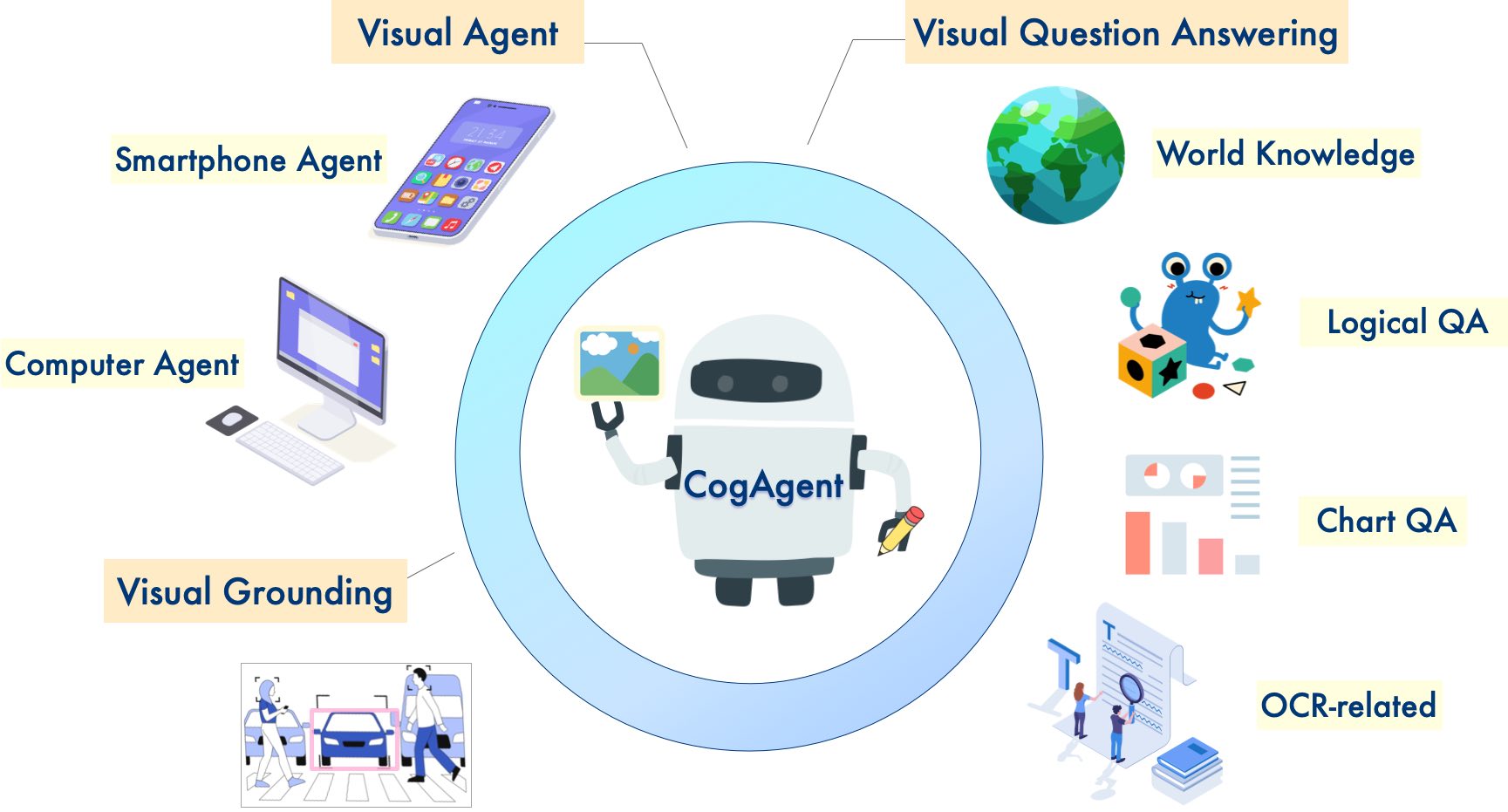
GUI Agent Examples

Cookbook
Task Prompts
-
General Multi-Round Dialogue: Say whatever you want.
-
GUI Agent Task: Use the Agent template and replace <TASK> with the task instruction enclosed in double quotes. This query can make CogAgent infer Plan and Next Action. If adding
(with grounding)
at the end of the query, the model will return a formalized action representation with coordinates.
For example, to ask the model how to complete the task "Search for CogVLM" on a current GUI screenshot, follow these steps:
-
Randomly select a template from the Agent template. Here, we choose
What steps do I need to take to <TASK>?
. -
Replace with the task instruction enclosed in double quotes, for example,
What steps do I need to take to "Search for CogVLM"?
. Inputting this to the model yields:
Plan: 1. Type 'CogVLM' into the Google search bar. 2. Review the search results that appear. 3. Click on a relevant result to read more about CogVLM or access further resources.
Next Action: Move the cursor to the Google search bar, and type 'CogVLM' into it.
- If adding
(with grounding)
at the end, i.e. changing the input toWhat steps do I need to take to "Search for CogVLM"?(with grounding)
, the output of CogAgent would be:
Plan: 1. Type 'CogVLM' into the Google search bar. 2. Review the search results that appear. 3. Click on a relevant result to read more about CogVLM or access further resources.
Next Action: Move the cursor to the Google search bar, and type 'CogVLM' into it. Grounded Operation:[combobox] Search -> TYPE: CogVLM at the box 212,498,787,564
Tip: For GUI Agent tasks, it is recommended to conduct only single-round dialogues for each image for better results.
-
Visual Grounding. Three modes of grounding are supported:
- Image description with grounding coordinates (bounding box). Use any template from caption_with_box template as model input. For example:
Can you provide a description of the image and include the coordinates x0,y0,x1,y1 for each mentioned object?
- Returning grounding coordinates (bounding box) based on the description of objects. Use any template from caption2box template, replacing
<expr>
with the object's description. For example:
Can you point out children in blue T-shirts in the image and provide the bounding boxes of their location?
- Providing a description based on bounding box coordinates. Use a template from box2caption template, replacing
<objs>
with the position coordinates. For example:
Tell me what you see within the designated area 086,540,400,760 in the picture.
Format of coordination: The bounding box coordinates in the model's input and output use the format [[x1, y1, x2, y2]]
, with the origin at the top left corner, the x-axis to the right, and the y-axis downward. (x1, y1) and (x2, y2) are the top-left and bottom-right corners, respectively, with values as relative coordinates multiplied by 1000 (prefixed with zeros to three digits).
Which --version to use
Due to differences in model functionalities, different model versions may have distinct --version
specifications for the text processor, meaning the format of the prompts used varies.
model name | --version |
---|---|
cogagent-chat | chat |
cogvlm-chat | chat_old |
cogvlm-chat-v1.1 | chat_old |
cogvlm-grounding-generalist | base |
cogvlm-base-224 | base |
cogvlm-base-490 | base |
FAQ
- If you have trouble in accessing huggingface.co, you can add
--local_tokenizer /path/to/vicuna-7b-v1.5
to load the tokenizer. - If you have trouble in automatically downloading model with 🔨SAT, try downloading from 🤖modelscope or 🤗huggingface or 💡wisemodel manually.
- Download model using 🔨SAT, the model will be saved to the default location
~/.sat_models
. Change the default location by setting the environment variableSAT_HOME
. For example, if you want to save the model to/path/to/my/models
, you can runexport SAT_HOME=/path/to/my/models
before running the python command.
License
The code in this repository is open source under the Apache-2.0 license, while the use of the CogVLM model weights must comply with the Model License.
Citation & Acknowledgements
If you find our work helpful, please consider citing the following papers
@misc{wang2023cogvlm,
title={CogVLM: Visual Expert for Pretrained Language Models},
author={Weihan Wang and Qingsong Lv and Wenmeng Yu and Wenyi Hong and Ji Qi and Yan Wang and Junhui Ji and Zhuoyi Yang and Lei Zhao and Xixuan Song and Jiazheng Xu and Bin Xu and Juanzi Li and Yuxiao Dong and Ming Ding and Jie Tang},
year={2023},
eprint={2311.03079},
archivePrefix={arXiv},
primaryClass={cs.CV}
}
@misc{hong2023cogagent,
title={CogAgent: A Visual Language Model for GUI Agents},
author={Wenyi Hong and Weihan Wang and Qingsong Lv and Jiazheng Xu and Wenmeng Yu and Junhui Ji and Yan Wang and Zihan Wang and Yuxiao Dong and Ming Ding and Jie Tang},
year={2023},
eprint={2312.08914},
archivePrefix={arXiv},
primaryClass={cs.CV}
}
In the instruction fine-tuning phase of the CogVLM, there are some English image-text data from the MiniGPT-4, LLAVA, LRV-Instruction, LLaVAR and Shikra projects, as well as many classic cross-modal work datasets. We sincerely thank them for their contributions.