mirror of https://github.com/open-mmlab/mmpose
1272 lines
93 KiB
Markdown
1272 lines
93 KiB
Markdown
<div align="center">
|
||
<img width="100%" src="https://github.com/open-mmlab/mmpose/assets/13503330/5b637d76-41dd-4376-9a7f-854cd120799d"/>
|
||
</div>
|
||
|
||
# RTMPose: Real-Time Multi-Person Pose Estimation toolkit based on MMPose
|
||
|
||
> [RTMPose: Real-Time Multi-Person Pose Estimation based on MMPose](https://arxiv.org/abs/2303.07399)
|
||
|
||
<div align="center">
|
||
|
||
[English](README.md) | 简体中文
|
||
|
||
</div>
|
||
|
||
______________________________________________________________________
|
||
|
||
## Abstract
|
||
|
||
近年来,2D 姿态估计的研究在公开数据集上取得了出色的成绩,但是它在工业界的应用仍然受到笨重的模型参数和高推理延迟的影响。为了让前沿姿态估计算法在工业界落地,我们通过实验研究了多人姿态估计算法的五个方面:范式、骨干网络、定位算法、训练策略和部署推理,基于 MMPose 提出了一个高性能的实时多人姿态估计框架 **RTMPose**。我们的 RTMPose-m 模型在 COCO 上取得 **75.8%AP**,在 Intel i7-11700 CPU 上达到 **90+FPS**,在 NVIDIA GTX 1660 Ti GPU 上达到 **430+FPS**。我们同样验证了在算力有限的设备上做实时姿态估计,RTMPose-s 在移动端骁龙865芯片上可以达到 **COCO 72.2%AP**,**70+FPS**。在 MMDeploy 的帮助下,我们的项目支持 CPU、GPU、Jetson、移动端等多种部署环境。
|
||
|
||
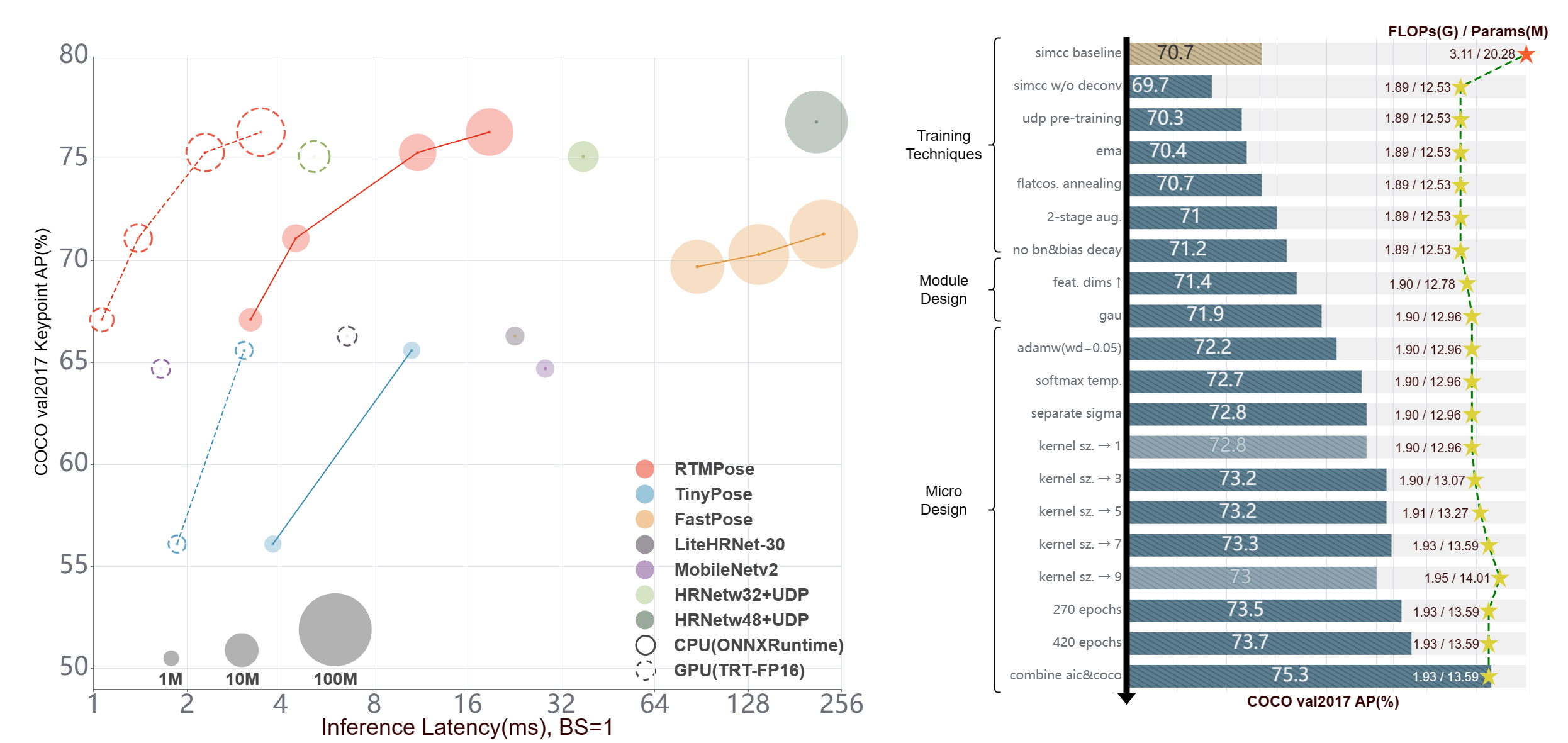
|
||
|
||
______________________________________________________________________
|
||
|
||
## 📄 Table of Contents
|
||
|
||
- [🥳 🚀 最新进展](#--最新进展-)
|
||
- [📖 简介](#-简介-)
|
||
- [🙌 社区共建](#-社区共建-)
|
||
- [⚡ Pipeline 性能](#-pipeline-性能-)
|
||
- [📊 模型库](#-模型库-)
|
||
- [👀 可视化](#-可视化-)
|
||
- [😎 快速尝试](#-快速尝试-)
|
||
- [👨🏫 模型训练](#-模型训练-)
|
||
- [🏗️ 部署教程](#️-部署教程-)
|
||
- [📚 常用功能](#️-常用功能-)
|
||
- [🚀 模型测速](#-模型测速-)
|
||
- [📊 精度验证](#-精度验证-)
|
||
- [📜 引用](#-引用-)
|
||
|
||
## 🥳 最新进展 [🔝](#-table-of-contents)
|
||
|
||
- 2023 年 12 月:
|
||
- 更新 RTMW 模型,RTMW-l 在 COCO-Wholebody 验证集上去的 70.1 mAP。
|
||
- 2023 年 9 月:
|
||
- 发布混合数据集上训练的 RTMW 模型。Alpha 版本的 RTMW-x 在 COCO-Wholebody 验证集上取得了 70.2 mAP。[在线 Demo](https://openxlab.org.cn/apps/detail/mmpose/RTMPose) 已支持 RTMW。技术报告正在撰写中。
|
||
- 增加 HumanArt 上训练的 YOLOX 和 RTMDet 模型。
|
||
- 2023 年 8 月:
|
||
- 支持基于 RTMPose 模型蒸馏的 133 点 WholeBody 模型(由 [DWPose](https://github.com/IDEA-Research/DWPose/tree/main) 提供)。
|
||
- 你可以在 [sd-webui-controlnet](https://github.com/Mikubill/sd-webui-controlnet) 中使用 DWPose/RTMPose 作为姿态估计后端进行人物图像生成。升级 sd-webui-controlnet >= v1.1237 并选择 `dw_openpose_full` 即可使用。
|
||
- [在线 Demo](https://openxlab.org.cn/apps/detail/mmpose/RTMPose) 已支持 DWPose,试玩请选择 `wholebody`。
|
||
- 2023 年 7 月:
|
||
- 在线 RTMPose 试玩 [Demo](https://openxlab.org.cn/apps/detail/mmpose/RTMPose)。
|
||
- 支持面向艺术图片人体姿态估计的 17 点 Body 模型。
|
||
- 2023 年 6 月:
|
||
- 发布混合数据集训练的 26 点 Body 模型。
|
||
- 2023 年 5 月:
|
||
- 已导出的 SDK 模型(ONNX、TRT、ncnn 等)可以从 [OpenMMLab Deploee](https://platform.openmmlab.com/deploee) 直接下载。
|
||
- [在线导出](https://platform.openmmlab.com/deploee/task-convert-list) SDK 模型(ONNX、TRT、ncnn 等)。
|
||
- 添加 [代码示例](./examples/),包括:
|
||
- 纯 Python 推理代码示例,无 MMDeploy、MMCV 依赖
|
||
- C++ 代码示例:ONNXRuntime、TensorRT
|
||
- Android 项目示例:基于 ncnn
|
||
- 发布混合数据集训练的 Hand, Face, Body 模型。
|
||
- 2023 年 3 月:发布 RTMPose。RTMPose-m 取得 COCO 验证集 75.8 mAP,推理速度达到 430+ FPS 。
|
||
|
||
## 📖 简介 [🔝](#-table-of-contents)
|
||
|
||
<div align=center>
|
||
<img src="https://user-images.githubusercontent.com/13503330/221138554-110240d8-e887-4b9a-90b1-2fbdc982e9de.gif" width=400 height=300/><img src="https://user-images.githubusercontent.com/13503330/221125176-85015a13-9648-4f0d-a17c-1cbb469efacf.gif" width=250 height=300/><img src="https://user-images.githubusercontent.com/13503330/221125310-7eeb2212-907e-427f-97af-af799d70a4c5.gif" width=250 height=300/>
|
||
</div>
|
||
|
||
<div align=center>
|
||
<img src="https://github.com/open-mmlab/mmpose/assets/13503330/38aa345e-4ceb-4e73-bc37-5e082735e336" width=450 height=300/><img src="https://user-images.githubusercontent.com/13503330/221125888-15c20faf-0ad5-4afb-828b-a71ccb064582.gif" width=450 height=300/>
|
||
</div>
|
||
<div align=center>
|
||
<img src="https://github.com/open-mmlab/mmpose/assets/13503330/2ecbf9f4-6963-4a14-9801-da10c0a65dac" width=300 height=350/><img src="https://user-images.githubusercontent.com/13503330/221138017-10431ab4-e515-4c32-8fa7-8748e2d17a58.gif" width=600 height=350/>
|
||
</div>
|
||
|
||
### ✨ 主要特性
|
||
|
||
- 🚀 **高精度,低延迟**
|
||
|
||
| Model | AP(COCO) | CPU-FPS | GPU-FPS |
|
||
| :---: | :------: | :-----: | :-----: |
|
||
| t | 68.5 | 300+ | 940+ |
|
||
| s | 72.2 | 200+ | 710+ |
|
||
| m | 75.8 | 90+ | 430+ |
|
||
| l | 76.5 | 50+ | 280+ |
|
||
| l-384 | 78.3 | - | 160+ |
|
||
|
||
- 🛠️ **易部署**
|
||
|
||
- 详细的部署代码教程,手把手教你模型部署
|
||
- MMDeploy 助力
|
||
- 支持多种部署后端
|
||
- ONNX
|
||
- TensorRT
|
||
- ncnn
|
||
- OpenVINO 等
|
||
- 支持多种平台
|
||
- Linux
|
||
- Windows
|
||
- NVIDIA Jetson
|
||
- ARM 等
|
||
|
||
- 🏗️ **为实际业务设计**
|
||
|
||
- 提供多种 Pipeline 推理接口和 SDK
|
||
- Python
|
||
- C++
|
||
- C#
|
||
- JAVA 等
|
||
|
||
## 🙌 社区共建 [🔝](#-table-of-contents)
|
||
|
||
RTMPose 是一个长期优化迭代的项目,致力于业务场景下的高性能实时姿态估计算法的训练、优化和部署,因此我们十分期待来自社区的力量,欢迎分享不同业务场景中 RTMPose 的训练配置与技巧,助力更多的社区用户!
|
||
|
||
✨ ✨ ✨
|
||
|
||
- **如果你是 RTMPose 的新用户,我们热切希望你能参与[这份问卷](https://uua478.fanqier.cn/f/xxmynrki)/[Google Questionnaire](https://docs.google.com/forms/d/e/1FAIpQLSfzwWr3eNlDzhU98qzk2Eph44Zio6hi5r0iSwfO9wSARkHdWg/viewform?usp=sf_link),这对于我们的工作非常重要!**
|
||
|
||
✨ ✨ ✨
|
||
|
||
欢迎加入我们的社区交流群获得更多帮助:
|
||
|
||
- 微信用户群
|
||
|
||
<div align=left>
|
||
<img src="https://user-images.githubusercontent.com/13503330/222647056-875bed70-85ec-455c-9016-c024772915c4.jpg" width=200 />
|
||
|
||
- Discord Group:
|
||
- 🙌 https://discord.gg/raweFPmdzG 🙌
|
||
|
||
## ⚡ Pipeline 性能 [🔝](#-table-of-contents)
|
||
|
||
**说明**
|
||
|
||
- Pipeline 速度测试时开启了隔帧检测策略,默认检测间隔为 5 帧。
|
||
- 环境配置:
|
||
- torch >= 1.7.1
|
||
- onnxruntime 1.12.1
|
||
- TensorRT 8.4.3.1
|
||
- cuDNN 8.3.2
|
||
- CUDA 11.3
|
||
- **更新**:我们推荐你使用混合数据集训练的 `Body8` 模型,性能高于下表中提供的模型,[传送门](#人体-2d-关键点)。
|
||
|
||
| Detection Config | Pose Config | Input Size<sup><br>(Det/Pose) | Model AP<sup><br>(COCO) | Pipeline AP<sup><br>(COCO) | Params (M)<sup><br>(Det/Pose) | Flops (G)<sup><br>(Det/Pose) | ORT-Latency(ms)<sup><br>(i7-11700) | TRT-FP16-Latency(ms)<sup><br>(GTX 1660Ti) | Download |
|
||
| :------------------------------------------------------------------ | :---------------------------------------------------------------------------- | :---------------------------: | :---------------------: | :------------------------: | :---------------------------: | :--------------------------: | :--------------------------------: | :---------------------------------------: | :------------------------------------------------------------------------------------------------------------------------------------------------------------------------------------------------------------------------------------------------------------------------: |
|
||
| [RTMDet-nano](./rtmdet/person/rtmdet_nano_320-8xb32_coco-person.py) | [RTMPose-t](./rtmpose/body_2d_keypoint/rtmpose-t_8xb256-420e_coco-256x192.py) | 320x320<br>256x192 | 40.3<br>67.1 | 64.4 | 0.99<br/>3.34 | 0.31<br/>0.36 | 12.403 | 2.467 | [det](https://download.openmmlab.com/mmpose/v1/projects/rtmpose/rtmdet_nano_8xb32-100e_coco-obj365-person-05d8511e.pth)<br/>[pose](https://download.openmmlab.com/mmpose/v1/projects/rtmposev1/rtmpose-tiny_simcc-aic-coco_pt-aic-coco_420e-256x192-cfc8f33d_20230126.pth) |
|
||
| [RTMDet-nano](./rtmdet/person/rtmdet_nano_320-8xb32_coco-person.py) | [RTMPose-s](./rtmpose/body_2d_keypoint/rtmpose-s_8xb256-420e_coco-256x192.py) | 320x320<br>256x192 | 40.3<br>71.1 | 68.5 | 0.99<br/>5.47 | 0.31<br/>0.68 | 16.658 | 2.730 | [det](https://download.openmmlab.com/mmpose/v1/projects/rtmpose/rtmdet_nano_8xb32-100e_coco-obj365-person-05d8511e.pth)<br/>[pose](https://download.openmmlab.com/mmpose/v1/projects/rtmposev1/rtmpose-s_simcc-aic-coco_pt-aic-coco_420e-256x192-fcb2599b_20230126.pth) |
|
||
| [RTMDet-nano](./rtmdet/person/rtmdet_nano_320-8xb32_coco-person.py) | [RTMPose-m](./rtmpose/body_2d_keypoint/rtmpose-m_8xb256-420e_coco-256x192.py) | 320x320<br>256x192 | 40.3<br>75.3 | 73.2 | 0.99<br/>13.59 | 0.31<br/>1.93 | 26.613 | 4.312 | [det](https://download.openmmlab.com/mmpose/v1/projects/rtmpose/rtmdet_nano_8xb32-100e_coco-obj365-person-05d8511e.pth)<br/>[pose](https://download.openmmlab.com/mmpose/v1/projects/rtmposev1/rtmpose-m_simcc-aic-coco_pt-aic-coco_420e-256x192-63eb25f7_20230126.pth) |
|
||
| [RTMDet-nano](./rtmdet/person/rtmdet_nano_320-8xb32_coco-person.py) | [RTMPose-l](./rtmpose/body_2d_keypoint/rtmpose-l_8xb256-420e_coco-256x192.py) | 320x320<br>256x192 | 40.3<br>76.3 | 74.2 | 0.99<br/>27.66 | 0.31<br/>4.16 | 36.311 | 4.644 | [det](https://download.openmmlab.com/mmpose/v1/projects/rtmpose/rtmdet_nano_8xb32-100e_coco-obj365-person-05d8511e.pth)<br/>[pose](https://download.openmmlab.com/mmpose/v1/projects/rtmposev1/rtmpose-l_simcc-aic-coco_pt-aic-coco_420e-256x192-f016ffe0_20230126.pth) |
|
||
| [RTMDet-m](./rtmdet/person/rtmdet_m_640-8xb32_coco-person.py) | [RTMPose-m](./rtmpose/body_2d_keypoint/rtmpose-m_8xb256-420e_coco-256x192.py) | 640x640<br>256x192 | 62.5<br>75.3 | 75.7 | 24.66<br/>13.59 | 38.95<br/>1.93 | - | 6.923 | [det](https://download.openmmlab.com/mmpose/v1/projects/rtmpose/rtmdet_m_8xb32-100e_coco-obj365-person-235e8209.pth)<br/>[pose](https://download.openmmlab.com/mmpose/v1/projects/rtmposev1/rtmpose-m_simcc-aic-coco_pt-aic-coco_420e-256x192-63eb25f7_20230126.pth) |
|
||
| [RTMDet-m](./rtmdet/person/rtmdet_m_640-8xb32_coco-person.py) | [RTMPose-l](./rtmpose/body_2d_keypoint/rtmpose-l_8xb256-420e_coco-256x192.py) | 640x640<br>256x192 | 62.5<br>76.3 | 76.6 | 24.66<br/>27.66 | 38.95<br/>4.16 | - | 7.204 | [det](https://download.openmmlab.com/mmpose/v1/projects/rtmpose/rtmdet_m_8xb32-100e_coco-obj365-person-235e8209.pth)<br/>[pose](https://download.openmmlab.com/mmpose/v1/projects/rtmposev1/rtmpose-l_simcc-aic-coco_pt-aic-coco_420e-256x192-f016ffe0_20230126.pth) |
|
||
|
||
## 📊 模型库 [🔝](#-table-of-contents)
|
||
|
||
**说明**
|
||
|
||
- 此处提供的模型采用了多数据集联合训练以提高性能,模型指标不适用于学术比较。
|
||
- 表格中为开启了 Flip Test 的测试结果。
|
||
- RTMPose 在更多公开数据集上的性能指标可以前往 [Model Zoo](https://mmpose.readthedocs.io/en/latest/model_zoo_papers/algorithms.html) 查看。
|
||
- RTMPose 在更多硬件平台上的推理速度可以前往 [Benchmark](./benchmark/README_CN.md) 查看。
|
||
- 如果你有希望我们支持的数据集,欢迎[联系我们](https://uua478.fanqier.cn/f/xxmynrki)/[Google Questionnaire](https://docs.google.com/forms/d/e/1FAIpQLSfzwWr3eNlDzhU98qzk2Eph44Zio6hi5r0iSwfO9wSARkHdWg/viewform?usp=sf_link)!
|
||
|
||
### 人体 2d 关键点
|
||
|
||
#### 17 Keypoints
|
||
|
||
- 关键点骨架定义遵循 [COCO](http://cocodataset.org/). 详情见 [meta info](/configs/_base_/datasets/coco.py).
|
||
- <img src="https://github.com/open-mmlab/mmpose/assets/13503330/2417e4f7-2203-468f-bad0-e7a6a6bf8251" height="300px">
|
||
|
||
<details close>
|
||
<summary><b>AIC+COCO</b></summary>
|
||
|
||
| Config | Input Size | AP<sup><br>(COCO) | PCK@0.1<sup><br>(Body8) | AUC<sup><br>(Body8) | Params<sup><br>(M) | FLOPS<sup><br>(G) | ORT-Latency<sup><br>(ms)<sup><br>(i7-11700) | TRT-FP16-Latency<sup><br>(ms)<sup><br>(GTX 1660Ti) | ncnn-FP16-Latency<sup><br>(ms)<sup><br>(Snapdragon 865) | Download |
|
||
| :---------------------------------------------------------------------------: | :--------: | :---------------: | :---------------------: | :-----------------: | :----------------: | :---------------: | :-----------------------------------------: | :------------------------------------------------: | :-----------------------------------------------------: | :-------------------------------------------------------------------------------------------------------------------------------------------: |
|
||
| [RTMPose-t](./rtmpose/body_2d_keypoint/rtmpose-t_8xb256-420e_coco-256x192.py) | 256x192 | 68.5 | 91.28 | 63.38 | 3.34 | 0.36 | 3.20 | 1.06 | 9.02 | [pth](https://download.openmmlab.com/mmpose/v1/projects/rtmposev1/rtmpose-tiny_simcc-aic-coco_pt-aic-coco_420e-256x192-cfc8f33d_20230126.pth) |
|
||
| [RTMPose-s](./rtmpose/body_2d_keypoint/rtmpose-s_8xb256-420e_coco-256x192.py) | 256x192 | 72.2 | 92.95 | 66.19 | 5.47 | 0.68 | 4.48 | 1.39 | 13.89 | [pth](https://download.openmmlab.com/mmpose/v1/projects/rtmposev1/rtmpose-s_simcc-aic-coco_pt-aic-coco_420e-256x192-fcb2599b_20230126.pth) |
|
||
| [RTMPose-m](./rtmpose/body_2d_keypoint/rtmpose-m_8xb256-420e_coco-256x192.py) | 256x192 | 75.8 | 94.13 | 68.53 | 13.59 | 1.93 | 11.06 | 2.29 | 26.44 | [pth](https://download.openmmlab.com/mmpose/v1/projects/rtmposev1/rtmpose-m_simcc-aic-coco_pt-aic-coco_420e-256x192-63eb25f7_20230126.pth) |
|
||
| [RTMPose-l](./rtmpose/body_2d_keypoint/rtmpose-l_8xb256-420e_coco-256x192.py) | 256x192 | 76.5 | 94.35 | 68.98 | 27.66 | 4.16 | 18.85 | 3.46 | 45.37 | [pth](https://download.openmmlab.com/mmpose/v1/projects/rtmposev1/rtmpose-l_simcc-aic-coco_pt-aic-coco_420e-256x192-f016ffe0_20230126.pth) |
|
||
| [RTMPose-m](./rtmpose/body_2d_keypoint/rtmpose-m_8xb256-420e_coco-384x288.py) | 384x288 | 77.0 | 94.32 | 69.85 | 13.72 | 4.33 | 24.78 | 3.66 | - | [pth](https://download.openmmlab.com/mmpose/v1/projects/rtmposev1/rtmpose-m_simcc-aic-coco_pt-aic-coco_420e-384x288-a62a0b32_20230228.pth) |
|
||
| [RTMPose-l](./rtmpose/body_2d_keypoint/rtmpose-l_8xb256-420e_coco-384x288.py) | 384x288 | 77.3 | 94.54 | 70.14 | 27.79 | 9.35 | - | 6.05 | - | [pth](https://download.openmmlab.com/mmpose/v1/projects/rtmposev1/rtmpose-l_simcc-aic-coco_pt-aic-coco_420e-384x288-97d6cb0f_20230228.pth) |
|
||
|
||
</details>
|
||
|
||
<details open>
|
||
<summary><b>Body8</b></summary>
|
||
|
||
- `*` 代表模型在 7 个开源数据集上训练得到:
|
||
- [AI Challenger](https://mmpose.readthedocs.io/en/latest/dataset_zoo/2d_body_keypoint.html#aic)
|
||
- [MS COCO](https://mmpose.readthedocs.io/en/latest/dataset_zoo/2d_body_keypoint.html#coco)
|
||
- [CrowdPose](https://mmpose.readthedocs.io/en/latest/dataset_zoo/2d_body_keypoint.html#crowdpose)
|
||
- [MPII](https://mmpose.readthedocs.io/en/latest/dataset_zoo/2d_body_keypoint.html#mpii)
|
||
- [sub-JHMDB](https://mmpose.readthedocs.io/en/latest/dataset_zoo/2d_body_keypoint.html#sub-jhmdb-dataset)
|
||
- [Halpe](https://mmpose.readthedocs.io/en/latest/dataset_zoo/2d_wholebody_keypoint.html#halpe)
|
||
- [PoseTrack18](https://mmpose.readthedocs.io/en/latest/dataset_zoo/2d_body_keypoint.html#posetrack18)
|
||
- `Body8` 代表除了以上提到的 7 个数据集,再加上 [OCHuman](https://mmpose.readthedocs.io/en/latest/dataset_zoo/2d_body_keypoint.html#ochuman) 合并后一起进行评测得到的指标。
|
||
|
||
| Config | Input Size | AP<sup><br>(COCO) | PCK@0.1<sup><br>(Body8) | AUC<sup><br>(Body8) | Params<sup><br>(M) | FLOPS<sup><br>(G) | ORT-Latency<sup><br>(ms)<sup><br>(i7-11700) | TRT-FP16-Latency<sup><br>(ms)<sup><br>(GTX 1660Ti) | ncnn-FP16-Latency<sup><br>(ms)<sup><br>(Snapdragon 865) | Download |
|
||
| :-----------------------------------------------------------------------------: | :--------: | :---------------: | :---------------------: | :-----------------: | :----------------: | :---------------: | :-----------------------------------------: | :------------------------------------------------: | :-----------------------------------------------------: | :------------------------------------------------------------------------------------------------------------------------------------------------------------------------------------------------------------------------------------------------------------------------------------: |
|
||
| [RTMPose-t\*](./rtmpose/body_2d_keypoint/rtmpose-t_8xb256-420e_coco-256x192.py) | 256x192 | 65.9 | 91.44 | 63.18 | 3.34 | 0.36 | 3.20 | 1.06 | 9.02 | [pth](https://download.openmmlab.com/mmpose/v1/projects/rtmposev1/rtmpose-t_simcc-body7_pt-body7_420e-256x192-026a1439_20230504.pth)<br>[onnx](https://download.openmmlab.com/mmpose/v1/projects/rtmposev1/onnx_sdk/rtmpose-t_simcc-body7_pt-body7_420e-256x192-026a1439_20230504.zip) |
|
||
| [RTMPose-s\*](./rtmpose/body_2d_keypoint/rtmpose-s_8xb256-420e_coco-256x192.py) | 256x192 | 69.7 | 92.45 | 65.15 | 5.47 | 0.68 | 4.48 | 1.39 | 13.89 | [pth](https://download.openmmlab.com/mmpose/v1/projects/rtmposev1/rtmpose-s_simcc-body7_pt-body7_420e-256x192-acd4a1ef_20230504.pth)<br>[onnx](https://download.openmmlab.com/mmpose/v1/projects/rtmposev1/onnx_sdk/rtmpose-s_simcc-body7_pt-body7_420e-256x192-acd4a1ef_20230504.zip) |
|
||
| [RTMPose-m\*](./rtmpose/body_2d_keypoint/rtmpose-m_8xb256-420e_coco-256x192.py) | 256x192 | 74.9 | 94.25 | 68.59 | 13.59 | 1.93 | 11.06 | 2.29 | 26.44 | [pth](https://download.openmmlab.com/mmpose/v1/projects/rtmposev1/rtmpose-m_simcc-body7_pt-body7_420e-256x192-e48f03d0_20230504.pth)<br>[onnx](https://download.openmmlab.com/mmpose/v1/projects/rtmposev1/onnx_sdk/rtmpose-m_simcc-body7_pt-body7_420e-256x192-e48f03d0_20230504.zip) |
|
||
| [RTMPose-l\*](./rtmpose/body_2d_keypoint/rtmpose-l_8xb256-420e_coco-256x192.py) | 256x192 | 76.7 | 95.08 | 70.14 | 27.66 | 4.16 | 18.85 | 3.46 | 45.37 | [pth](https://download.openmmlab.com/mmpose/v1/projects/rtmposev1/rtmpose-l_simcc-body7_pt-body7_420e-256x192-4dba18fc_20230504.pth)<br>[onnx](https://download.openmmlab.com/mmpose/v1/projects/rtmposev1/onnx_sdk/rtmpose-l_simcc-body7_pt-body7_420e-256x192-4dba18fc_20230504.zip) |
|
||
| [RTMPose-m\*](./rtmpose/body_2d_keypoint/rtmpose-m_8xb256-420e_coco-384x288.py) | 384x288 | 76.6 | 94.64 | 70.38 | 13.72 | 4.33 | 24.78 | 3.66 | - | [pth](https://download.openmmlab.com/mmpose/v1/projects/rtmposev1/rtmpose-m_simcc-body7_pt-body7_420e-384x288-65e718c4_20230504.pth)<br>[onnx](https://download.openmmlab.com/mmpose/v1/projects/rtmposev1/onnx_sdk/rtmpose-m_simcc-body7_pt-body7_420e-384x288-65e718c4_20230504.zip) |
|
||
| [RTMPose-l\*](./rtmpose/body_2d_keypoint/rtmpose-l_8xb256-420e_coco-384x288.py) | 384x288 | 78.3 | 95.36 | 71.58 | 27.79 | 9.35 | - | 6.05 | - | [pth](https://download.openmmlab.com/mmpose/v1/projects/rtmposev1/rtmpose-l_simcc-body7_pt-body7_420e-384x288-3f5a1437_20230504.pth)<br>[onnx](https://download.openmmlab.com/mmpose/v1/projects/rtmposev1/onnx_sdk/rtmpose-l_simcc-body7_pt-body7_420e-384x288-3f5a1437_20230504.zip) |
|
||
| [RTMPose-x\*](./rtmpose/body_2d_keypoint/rtmpose-x_8xb256-700e_coco-384x288.py) | 384x288 | 78.8 | - | - | 49.43 | 17.22 | - | - | - | [pth](https://download.openmmlab.com/mmpose/v1/projects/rtmposev1/rtmpose-x_simcc-body7_pt-body7_700e-384x288-71d7b7e9_20230629.pth)<br>[onnx](https://download.openmmlab.com/mmpose/v1/projects/rtmposev1/onnx_sdk/rtmpose-x_simcc-body7_pt-body7_700e-384x288-71d7b7e9_20230629.zip) |
|
||
|
||
</details>
|
||
|
||
<details open>
|
||
<summary><b>Human-Art</b></summary>
|
||
|
||
- 面向艺术图片的人体姿态估计 RTMPose 模型由 [Human-Art](https://github.com/IDEA-Research/HumanArt) 提供。
|
||
- <img src="https://github.com/open-mmlab/mmpose/assets/13503330/685bc610-dd9e-4e6f-9c41-dbc8220584f4" height="300px">
|
||
|
||
人体检测模型:
|
||
|
||
| Detection Config | Input Size | Model AP<sup><br>(OneHand10K) | Flops<sup><br>(G) | ORT-Latency<sup><br>(ms)<sup><br>(i7-11700) | TRT-FP16-Latency<sup><br>(ms)<sup><br>(GTX 1660Ti) | Download |
|
||
| :---------------------------: | :--------: | :---------------------------: | :---------------: | :-----------------------------------------: | :------------------------------------------------: | :--------------------: |
|
||
| [RTMDet-tiny](./rtmdet/person/rtmdet_tiny_8xb32-300e_humanart.py) | 640x640 | 46.6 | - | - | - | [Det Model](https://download.openmmlab.com/mmpose/v1/projects/rtmposev1/rtmdet_tiny_8xb32-300e_humanart-7da5554e.pth) |
|
||
| [RTMDet-s](./rtmdet/person/rtmdet_s_8xb32-300e_humanart.py) | 640x640 | 50.6 | - | - | - | [Det Model](https://download.openmmlab.com/mmpose/v1/projects/rtmposev1/rtmdet_s_8xb32-300e_humanart-af5bd52d.pth) |
|
||
| [YOLOX-nano](./yolox/humanart/yolox_nano_8xb8-300e_humanart.py) | 640x640 | 38.9 | - | - | - | [Det Model](https://download.openmmlab.com/mmpose/v1/projects/rtmposev1/yolox_nano_8xb8-300e_humanart-40f6f0d0.pth) |
|
||
| [YOLOX-tiny](./yolox/humanart/yolox_tiny_8xb8-300e_humanart.py) | 640x640 | 47.7 | - | - | - | [Det Model](https://download.openmmlab.com/mmpose/v1/projects/rtmposev1/yolox_tiny_8xb8-300e_humanart-6f3252f9.pth) |
|
||
| [YOLOX-s](./yolox/humanart/yolox_s_8xb8-300e_humanart.py) | 640x640 | 54.6 | - | - | - | [Det Model](https://download.openmmlab.com/mmpose/v1/projects/rtmposev1/yolox_s_8xb8-300e_humanart-3ef259a7.pth) |
|
||
| [YOLOX-m](./yolox/humanart/yolox_m_8xb8-300e_humanart.py) | 640x640 | 59.1 | - | - | - | [Det Model](https://download.openmmlab.com/mmpose/v1/projects/rtmposev1/yolox_m_8xb8-300e_humanart-c2c7a14a.pth) |
|
||
| [YOLOX-l](./yolox/humanart/yolox_l_8xb8-300e_humanart.py) | 640x640 | 60.2 | - | - | - | [Det Model](https://download.openmmlab.com/mmpose/v1/projects/rtmposev1/yolox_l_8xb8-300e_humanart-ce1d7a62.pth) |
|
||
| [YOLOX-x](./yolox/humanart/yolox_x_8xb8-300e_humanart.py) | 640x640 | 61.3 | - | - | - | [Det Model](https://download.openmmlab.com/mmpose/v1/projects/rtmposev1/yolox_x_8xb8-300e_humanart-a39d44ed.pth) |
|
||
|
||
人体姿态估计模型:
|
||
|
||
| Config | Input Size | AP<sup><br>(Human-Art GT) | Params<sup><br>(M) | FLOPS<sup><br>(G) | ORT-Latency<sup><br>(ms)<sup><br>(i7-11700) | TRT-FP16-Latency<sup><br>(ms)<sup><br>(GTX 1660Ti) | ncnn-FP16-Latency<sup><br>(ms)<sup><br>(Snapdragon 865) | Download |
|
||
| :-----------------------------------------------------------------------------: | :--------: | :-----------------------: | :----------------: | :---------------: | :-----------------------------------------: | :------------------------------------------------: | :-----------------------------------------------------: | :--------------------------------------------------------------------------------------------------------------------------------------------------------------------------------------------------------------------------------------------------------------------------: |
|
||
| [RTMPose-t\*](./rtmpose/body_2d_keypoint/rtmpose-t_8xb256-420e_coco-256x192.py) | 256x192 | 65.5 | 3.34 | 0.36 | 3.20 | 1.06 | 9.02 | [pth](https://download.openmmlab.com/mmpose/v1/projects/rtmposev1/rtmpose-t_8xb256-420e_humanart-256x192-60b68c98_20230612.pth)<br>[onnx](https://download.openmmlab.com/mmpose/v1/projects/rtmposev1/onnx_sdk/rtmpose-t_8xb256-420e_humanart-256x192-60b68c98_20230612.zip) |
|
||
| [RTMPose-s\*](./rtmpose/body_2d_keypoint/rtmpose-s_8xb256-420e_coco-256x192.py) | 256x192 | 69.8 | 5.47 | 0.68 | 4.48 | 1.39 | 13.89 | [pth](https://download.openmmlab.com/mmpose/v1/projects/rtmposev1/rtmpose-s_8xb256-420e_humanart-256x192-5a3ac943_20230611.pth)<br>[onnx](https://download.openmmlab.com/mmpose/v1/projects/rtmposev1/onnx_sdk/rtmpose-s_8xb256-420e_humanart-256x192-5a3ac943_20230611.zip) |
|
||
| [RTMPose-m\*](./rtmpose/body_2d_keypoint/rtmpose-m_8xb256-420e_coco-256x192.py) | 256x192 | 72.8 | 13.59 | 1.93 | 11.06 | 2.29 | 26.44 | [pth](https://download.openmmlab.com/mmpose/v1/projects/rtmposev1/rtmpose-m_8xb256-420e_humanart-256x192-8430627b_20230611.pth)<br>[onnx](https://download.openmmlab.com/mmpose/v1/projects/rtmposev1/onnx_sdk/rtmpose-m_8xb256-420e_humanart-256x192-8430627b_20230611.zip) |
|
||
| [RTMPose-l\*](./rtmpose/body_2d_keypoint/rtmpose-l_8xb256-420e_coco-256x192.py) | 256x192 | 75.3 | 27.66 | 4.16 | 18.85 | 3.46 | 45.37 | [pth](https://download.openmmlab.com/mmpose/v1/projects/rtmposev1/rtmpose-l_8xb256-420e_humanart-256x192-389f2cb0_20230611.pth)<br>[onnx](https://download.openmmlab.com/mmpose/v1/projects/rtmposev1/onnx_sdk/rtmpose-l_8xb256-420e_humanart-256x192-389f2cb0_20230611.zip) |
|
||
|
||
</details>
|
||
|
||
#### 26 Keypoints
|
||
|
||
- 关键点骨架定义遵循 [Halpe26](https://github.com/Fang-Haoshu/Halpe-FullBody/),详情见 [meta info](/configs/_base_/datasets/halpe26.py)。
|
||
- <img src="https://github.com/open-mmlab/mmpose/assets/13503330/f28ab3ba-833d-4ca7-8739-f97e6cafbab7" height="300px">
|
||
- 模型在 `Body8` 上进行训练和评估。
|
||
|
||
| Config | Input Size | PCK@0.1<sup><br>(Body8) | AUC<sup><br>(Body8) | Params(M) | FLOPS(G) | ORT-Latency<sup><br>(ms)<sup><br>(i7-11700) | TRT-FP16-Latency<sup><br>(ms)<sup><br>(GTX 1660Ti) | ncnn-FP16-Latency<sup><br>(ms)<sup><br>(Snapdragon 865) | Download |
|
||
| :---------------------------------------------------------------------------------------: | :--------: | :---------------------: | :-----------------: | :-------: | :------: | :-----------------------------------------: | :------------------------------------------------: | :-----------------------------------------------------: | :----------------------------------------------------------------------------------------------------------------------------------------------------------------------------------------------------------------------------------------------------------------------------------------------------: |
|
||
| [RTMPose-t\*](./rtmpose/body_2d_keypoint/rtmpose-t_8xb1024-700e_body8-halpe26-256x192.py) | 256x192 | 91.89 | 66.35 | 3.51 | 0.37 | - | - | - | [pth](https://download.openmmlab.com/mmpose/v1/projects/rtmposev1/rtmpose-t_simcc-body7_pt-body7-halpe26_700e-256x192-6020f8a6_20230605.pth)<br>[onnx](https://download.openmmlab.com/mmpose/v1/projects/rtmposev1/onnx_sdk/rtmpose-t_simcc-body7_pt-body7-halpe26_700e-256x192-6020f8a6_20230605.zip) |
|
||
| [RTMPose-s\*](./rtmpose/body_2d_keypoint/rtmpose-s_8xb1024-700e_body8-halpe26-256x192.py) | 256x192 | 93.01 | 68.62 | 5.70 | 0.70 | - | - | - | [pth](https://download.openmmlab.com/mmpose/v1/projects/rtmposev1/rtmpose-s_simcc-body7_pt-body7-halpe26_700e-256x192-7f134165_20230605.pth)<br>[onnx](https://download.openmmlab.com/mmpose/v1/projects/rtmposev1/onnx_sdk/rtmpose-s_simcc-body7_pt-body7-halpe26_700e-256x192-7f134165_20230605.zip) |
|
||
| [RTMPose-m\*](./rtmpose/body_2d_keypoint/rtmpose-m_8xb512-700e_body8-halpe26-256x192.py) | 256x192 | 94.75 | 71.91 | 13.93 | 1.95 | - | - | - | [pth](https://download.openmmlab.com/mmpose/v1/projects/rtmposev1/rtmpose-m_simcc-body7_pt-body7-halpe26_700e-256x192-4d3e73dd_20230605.pth)<br>[onnx](https://download.openmmlab.com/mmpose/v1/projects/rtmposev1/onnx_sdk/rtmpose-m_simcc-body7_pt-body7-halpe26_700e-256x192-4d3e73dd_20230605.zip) |
|
||
| [RTMPose-l\*](./rtmpose/body_2d_keypoint/rtmpose-l_8xb512-700e_body8-halpe26-256x192.py) | 256x192 | 95.37 | 73.19 | 28.11 | 4.19 | - | - | - | [pth](https://download.openmmlab.com/mmpose/v1/projects/rtmposev1/rtmpose-l_simcc-body7_pt-body7-halpe26_700e-256x192-2abb7558_20230605.pth)<br>[onnx](https://download.openmmlab.com/mmpose/v1/projects/rtmposev1/onnx_sdk/rtmpose-l_simcc-body7_pt-body7-halpe26_700e-256x192-2abb7558_20230605.zip) |
|
||
| [RTMPose-m\*](./rtmpose/body_2d_keypoint/rtmpose-m_8xb512-700e_body8-halpe26-384x288.py) | 384x288 | 95.15 | 73.56 | 14.06 | 4.37 | - | - | - | [pth](https://download.openmmlab.com/mmpose/v1/projects/rtmposev1/rtmpose-m_simcc-body7_pt-body7-halpe26_700e-384x288-89e6428b_20230605.pth)<br>[onnx](https://download.openmmlab.com/mmpose/v1/projects/rtmposev1/onnx_sdk/rtmpose-m_simcc-body7_pt-body7-halpe26_700e-384x288-89e6428b_20230605.zip) |
|
||
| [RTMPose-l\*](./rtmpose/body_2d_keypoint/rtmpose-l_8xb512-700e_body8-halpe26-384x288.py) | 384x288 | 95.56 | 74.38 | 28.24 | 9.40 | - | - | - | [pth](https://download.openmmlab.com/mmpose/v1/projects/rtmposev1/rtmpose-l_simcc-body7_pt-body7-halpe26_700e-384x288-734182ce_20230605.pth)<br>[onnx](https://download.openmmlab.com/mmpose/v1/projects/rtmposev1/onnx_sdk/rtmpose-l_simcc-body7_pt-body7-halpe26_700e-384x288-734182ce_20230605.zip) |
|
||
| [RTMPose-x\*](./rtmpose/body_2d_keypoint/rtmpose-x_8xb256-700e_body8-halpe26-384x288.py) | 384x288 | 95.74 | 74.82 | 50.00 | 17.29 | - | - | - | [pth](https://download.openmmlab.com/mmpose/v1/projects/rtmposev1/rtmpose-x_simcc-body7_pt-body7-halpe26_700e-384x288-7fb6e239_20230606.pth)<br>[onnx](https://download.openmmlab.com/mmpose/v1/projects/rtmposev1/onnx_sdk/rtmpose-x_simcc-body7_pt-body7-halpe26_700e-384x288-7fb6e239_20230606.zip) |
|
||
|
||
#### 模型剪枝
|
||
|
||
**说明**
|
||
|
||
- 模型剪枝由 [MMRazor](https://github.com/open-mmlab/mmrazor) 提供
|
||
|
||
| Config | Input Size | AP<sup><br>(COCO) | Params<sup><br>(M) | FLOPS<sup><br>(G) | ORT-Latency<sup><br>(ms)<sup><br>(i7-11700) | TRT-FP16-Latency<sup><br>(ms)<sup><br>(GTX 1660Ti) | ncnn-FP16-Latency<sup><br>(ms)<sup><br>(Snapdragon 865) | Download |
|
||
| :-----------------------: | :--------: | :---------------: | :----------------: | :---------------: | :-----------------------------------------: | :------------------------------------------------: | :-----------------------------------------------------: | :----------------------------------------------------------------------------------------------------------------------------------------------: |
|
||
| RTMPose-s-aic-coco-pruned | 256x192 | 69.4 | 3.43 | 0.35 | - | - | - | [pth](https://download.openmmlab.com/mmrazor/v1/pruning/group_fisher/rtmpose-s/group_fisher_finetune_rtmpose-s_8xb256-420e_aic-coco-256x192.pth) |
|
||
|
||
更多信息,请参考 [GroupFisher Pruning for RTMPose](./rtmpose/pruning/README.md).
|
||
|
||
### 人体全身 2d 关键点 (133 Keypoints)
|
||
|
||
- 关键点骨架定义遵循 [COCO-WholeBody](https://github.com/jin-s13/COCO-WholeBody/),详情见 [meta info](/configs/_base_/datasets/coco_wholebody.py)。
|
||
- <img src="https://user-images.githubusercontent.com/100993824/227770977-c8f00355-c43a-467e-8444-d307789cf4b2.png" height="300px">
|
||
|
||
<details close>
|
||
<summary><b>COCO-WholeBody</b></summary>
|
||
|
||
| Config | Input Size | Whole AP | Whole AR | FLOPS<sup><br>(G) | ORT-Latency<sup><br>(ms)<sup><br>(i7-11700) | TRT-FP16-Latency<sup><br>(ms)<sup><br>(GTX 1660Ti) | Download |
|
||
| :------------------------------ | :--------: | :------: | :------: | :---------------: | :-----------------------------------------: | :------------------------------------------------: | :-------------------------------: |
|
||
| [RTMPose-m](./rtmpose/wholebody_2d_keypoint/rtmpose-m_8xb64-270e_coco-wholebody-256x192.py) | 256x192 | 58.2 | 67.4 | 2.22 | 13.50 | 4.00 | [pth](https://download.openmmlab.com/mmpose/v1/projects/rtmposev1/rtmpose-m_simcc-coco-wholebody_pt-aic-coco_270e-256x192-cd5e845c_20230123.pth) |
|
||
| [RTMPose-l](./rtmpose/wholebody_2d_keypoint/rtmpose-l_8xb64-270e_coco-wholebody-256x192.py) | 256x192 | 61.1 | 70.0 | 4.52 | 23.41 | 5.67 | [pth](https://download.openmmlab.com/mmpose/v1/projects/rtmposev1/rtmpose-l_simcc-coco-wholebody_pt-aic-coco_270e-256x192-6f206314_20230124.pth) |
|
||
| [RTMPose-l](./rtmpose/wholebody_2d_keypoint/rtmpose-l_8xb32-270e_coco-wholebody-384x288.py) | 384x288 | 64.8 | 73.0 | 10.07 | 44.58 | 7.68 | [pth](https://download.openmmlab.com/mmpose/v1/projects/rtmposev1/rtmpose-l_simcc-coco-wholebody_pt-aic-coco_270e-384x288-eaeb96c8_20230125.pth) |
|
||
| [RTMPose-x](./rtmpose/wholebody_2d_keypoint/rtmpose-x_8xb32-270e_coco-wholebody-384x288.py) | 384x288 | 65.3 | 73.3 | 18.1 | - | - | [pth](https://download.openmmlab.com/mmpose/v1/projects/rtmposev1/rtmpose-x_simcc-coco-wholebody_pt-body7_270e-384x288-401dfc90_20230629.pth) |
|
||
|
||
</details>
|
||
|
||
<details open>
|
||
<summary><b>Cocktail14</b></summary>
|
||
|
||
- `Cocktail13` 代表模型在 13 个开源数据集上训练得到:
|
||
- [AI Challenger](https://mmpose.readthedocs.io/en/latest/dataset_zoo/2d_body_keypoint.html#aic)
|
||
- [CrowdPose](https://mmpose.readthedocs.io/en/latest/dataset_zoo/2d_body_keypoint.html#crowdpose)
|
||
- [MPII](https://mmpose.readthedocs.io/en/latest/dataset_zoo/2d_body_keypoint.html#mpii)
|
||
- [sub-JHMDB](https://mmpose.readthedocs.io/en/latest/dataset_zoo/2d_body_keypoint.html#sub-jhmdb-dataset)
|
||
- [Halpe](https://mmpose.readthedocs.io/en/latest/dataset_zoo/2d_wholebody_keypoint.html#halpe)
|
||
- [PoseTrack18](https://mmpose.readthedocs.io/en/latest/dataset_zoo/2d_body_keypoint.html#posetrack18)
|
||
- [COCO-Wholebody](https://github.com/jin-s13/COCO-WholeBody/)
|
||
- [UBody](https://github.com/IDEA-Research/OSX)
|
||
- [Human-Art](https://mmpose.readthedocs.io/en/latest/dataset_zoo/2d_body_keypoint.html#human-art-dataset)
|
||
- [WFLW](https://wywu.github.io/projects/LAB/WFLW.html)
|
||
- [300W](https://ibug.doc.ic.ac.uk/resources/300-W/)
|
||
- [COFW](http://www.vision.caltech.edu/xpburgos/ICCV13/)
|
||
- [LaPa](https://github.com/JDAI-CV/lapa-dataset)
|
||
- [InterHand](https://mks0601.github.io/InterHand2.6M/)
|
||
|
||
| Config | Input Size | Whole AP | Whole AR | FLOPS<sup><br>(G) | ORT-Latency<sup><br>(ms)<sup><br>(i7-11700) | TRT-FP16-Latency<sup><br>(ms)<sup><br>(GTX 1660Ti) | Download |
|
||
| :------------------------------ | :--------: | :------: | :------: | :---------------: | :-----------------------------------------: | :------------------------------------------------: | :-------------------------------: |
|
||
| [RTMW-m](./rtmpose/wholebody_2d_keypoint/rtmw-m_8xb1024-270e_cocktail14-256x192.py) | 256x192 | 58.2 | 67.3 | 4.3 | - | - | [pth](https://download.openmmlab.com/mmpose/v1/projects/rtmw/rtmw-dw-l-m_simcc-cocktail14_270e-256x192-20231122.pth)<br>[onnx](https://download.openmmlab.com/mmpose/v1/projects/rtmw/onnx_sdk/rtmw-dw-m-s_simcc-cocktail14_270e-256x192_20231122.zip) |
|
||
| [RTMW-l](./rtmpose/wholebody_2d_keypoint/rtmw-x_8xb704-270e_cocktail14-256x192.py) | 256x192 | 66.0 | 74.6 | 7.9 | - | - | [pth](https://download.openmmlab.com/mmpose/v1/projects/rtmw/rtmw-dw-x-l_simcc-cocktail14_270e-256x192-20231122.pth)<br>[onnx](https://download.openmmlab.com/mmpose/v1/projects/rtmw/onnx_sdk/rtmw-dw-x-l_simcc-cocktail14_270e-256x192_20231122.zip) |
|
||
| [RTMW-x](./rtmpose/wholebody_2d_keypoint/rtmw-x_8xb704-270e_cocktail14-256x192.py) | 256x192 | 67.2 | 75.2 | 13.1 | - | - | [pth](https://download.openmmlab.com/mmpose/v1/projects/rtmw/rtmw-x_simcc-cocktail14_pt-ucoco_270e-256x192-13a2546d_20231208.pth) |
|
||
| [RTMW-l](./rtmpose/wholebody_2d_keypoint/rtmw-x_8xb320-270e_cocktail14-384x288.py) | 384x288 | 70.1 | 78.0 | 17.7 | - | - | [pth](https://download.openmmlab.com/mmpose/v1/projects/rtmw/rtmw-dw-x-l_simcc-cocktail14_270e-384x288-20231122.pth)<br>[onnx](https://download.openmmlab.com/mmpose/v1/projects/rtmw/onnx_sdk/rtmw-dw-x-l_simcc-cocktail14_270e-384x288_20231122.zip) |
|
||
| [RTMW-x](./rtmpose/wholebody_2d_keypoint/rtmw-x_8xb320-270e_cocktail14-384x288.py) | 384x288 | 70.2 | 78.1 | 29.3 | - | - | [pth](https://download.openmmlab.com/mmpose/v1/projects/rtmw/rtmw-x_simcc-cocktail14_pt-ucoco_270e-384x288-f840f204_20231122.pth) |
|
||
|
||
</details>
|
||
|
||
<details open>
|
||
<summary><b>COCO+UBody</b></summary>
|
||
|
||
- DWPose 模型由 [DWPose](https://github.com/IDEA-Research/DWPose) 项目提供
|
||
- 模型在以下数据集上训练并蒸馏:
|
||
- [COCO-WholeBody](https://github.com/jin-s13/COCO-WholeBody/)
|
||
- [UBody](https://github.com/IDEA-Research/OSX)
|
||
|
||
| Config | Input Size | Whole AP | Whole AR | FLOPS<sup><br>(G) | ORT-Latency<sup><br>(ms)<sup><br>(i7-11700) | TRT-FP16-Latency<sup><br>(ms)<sup><br>(GTX 1660Ti) | Download |
|
||
| :------------------------------ | :--------: | :------: | :------: | :---------------: | :-----------------------------------------: | :------------------------------------------------: | :-------------------------------: |
|
||
| [RTMPose-t](./rtmpose/wholebody_2d_keypoint/rtmpose-t_8xb64-270e_coco-wholebody-256x192.py) | 256x192 | 48.5 | 58.4 | 0.5 | - | - | [pth](https://download.openmmlab.com/mmpose/v1/projects/rtmposev1/rtmpose-t_simcc-ucoco_dw-ucoco_270e-256x192-dcf277bf_20230728.pth)<br>[onnx](https://download.openmmlab.com/mmpose/v1/projects/rtmposev1/onnx_sdk/rtmpose-t_simcc-ucoco_dw-ucoco_270e-256x192-dcf277bf_20230728.zip) |
|
||
| [RTMPose-s](./rtmpose/wholebody_2d_keypoint/rtmpose-s_8xb64-270e_coco-wholebody-256x192.py) | 256x192 | 53.8 | 63.2 | 0.9 | - | - | [pth](https://download.openmmlab.com/mmpose/v1/projects/rtmposev1/rtmpose-s_simcc-ucoco_dw-ucoco_270e-256x192-3fd922c8_20230728.pth)<br>[onnx](https://download.openmmlab.com/mmpose/v1/projects/rtmposev1/onnx_sdk/rtmpose-s_simcc-ucoco_dw-ucoco_270e-256x192-3fd922c8_20230728.zip) |
|
||
| [RTMPose-m](./rtmpose/wholebody_2d_keypoint/rtmpose-m_8xb64-270e_coco-wholebody-256x192.py) | 256x192 | 60.6 | 69.5 | 2.22 | 13.50 | 4.00 | [pth](https://download.openmmlab.com/mmpose/v1/projects/rtmposev1/rtmpose-m_simcc-ucoco_dw-ucoco_270e-256x192-c8b76419_20230728.pth)<br>[onnx](https://download.openmmlab.com/mmpose/v1/projects/rtmposev1/onnx_sdk/rtmpose-m_simcc-ucoco_dw-ucoco_270e-256x192-c8b76419_20230728.zip) |
|
||
| [RTMPose-l](./rtmpose/wholebody_2d_keypoint/rtmpose-l_8xb64-270e_coco-wholebody-256x192.py) | 256x192 | 63.1 | 71.7 | 4.52 | 23.41 | 5.67 | [pth](https://download.openmmlab.com/mmpose/v1/projects/rtmposev1/rtmpose-l_simcc-ucoco_dw-ucoco_270e-256x192-4d6dfc62_20230728.pth)<br>[onnx](https://download.openmmlab.com/mmpose/v1/projects/rtmposev1/onnx_sdk/rtmpose-l_simcc-ucoco_dw-ucoco_270e-256x192-4d6dfc62_20230728.zip) |
|
||
| [RTMPose-l](./rtmpose/wholebody_2d_keypoint/rtmpose-l_8xb32-270e_coco-wholebody-384x288.py) | 384x288 | 66.5 | 74.3 | 10.07 | 44.58 | 7.68 | [pth](https://download.openmmlab.com/mmpose/v1/projects/rtmposev1/rtmpose-l_simcc-ucoco_dw-ucoco_270e-384x288-2438fd99_20230728.pth)<br>[onnx](https://download.openmmlab.com/mmpose/v1/projects/rtmposev1/onnx_sdk/rtmpose-l_simcc-ucoco_dw-ucoco_270e-384x288-2438fd99_20230728.zip) |
|
||
|
||
</details>
|
||
|
||
### 动物 2d 关键点 (17 Keypoints)
|
||
|
||
- 关键点骨架定义遵循 [AP-10K](https://github.com/AlexTheBad/AP-10K/),详情见 [meta info](/configs/_base_/datasets/ap10k.py)。
|
||
- <img src="https://user-images.githubusercontent.com/100993824/227797151-091dc21a-d944-49c9-8b62-cc47fa89e69f.png" height="300px">
|
||
|
||
| Config | Input Size | AP<sup><br>(AP10K) | FLOPS<sup><br>(G) | ORT-Latency<sup><br>(ms)<sup><br>(i7-11700) | TRT-FP16-Latency<sup><br>(ms)<sup><br>(GTX 1660Ti) | Download |
|
||
| :----------------------------: | :--------: | :----------------: | :---------------: | :-----------------------------------------: | :------------------------------------------------: | :------------------------------: |
|
||
| [RTMPose-m](./rtmpose/animal_2d_keypoint/rtmpose-m_8xb64-210e_ap10k-256x256.py) | 256x256 | 72.2 | 2.57 | 14.157 | 2.404 | [pth](https://download.openmmlab.com/mmpose/v1/projects/rtmposev1/rtmpose-m_simcc-ap10k_pt-aic-coco_210e-256x256-7a041aa1_20230206.pth)<br>[onnx](https://download.openmmlab.com/mmpose/v1/projects/rtmposev1/onnx_sdk/rtmpose-m_simcc-ap10k_pt-aic-coco_210e-256x256-7a041aa1_20230206.zip) |
|
||
|
||
### 脸部 2d 关键点 (106 Keypoints)
|
||
|
||
- 关键点骨架定义遵循 [LaPa](https://github.com/JDAI-CV/lapa-dataset),详情见 [meta info](/configs/_base_/datasets/lapa.py)。
|
||
- <img src="https://github.com/open-mmlab/mmpose/assets/13503330/30fa583e-500c-4356-ac5a-7e9d7d18381a" height="300px">
|
||
|
||
<details open>
|
||
<summary><b>Face6</b></summary>
|
||
|
||
- `Face6` and `*` 代表模型在 6 个开源数据集上训练得到:
|
||
- [COCO-Wholebody-Face](https://github.com/jin-s13/COCO-WholeBody/)
|
||
- [WFLW](https://wywu.github.io/projects/LAB/WFLW.html)
|
||
- [300W](https://ibug.doc.ic.ac.uk/resources/300-W/)
|
||
- [COFW](http://www.vision.caltech.edu/xpburgos/ICCV13/)
|
||
- [Halpe](https://github.com/Fang-Haoshu/Halpe-FullBody/)
|
||
- [LaPa](https://github.com/JDAI-CV/lapa-dataset)
|
||
|
||
| Config | Input Size | NME<sup><br>(LaPa) | FLOPS<sup><br>(G) | ORT-Latency<sup><br>(ms)<sup><br>(i7-11700) | TRT-FP16-Latency<sup><br>(ms)<sup><br>(GTX 1660Ti) | Download |
|
||
| :----------------------------: | :--------: | :----------------: | :---------------: | :-----------------------------------------: | :------------------------------------------------: | :------------------------------: |
|
||
| [RTMPose-t\*](./rtmpose/face_2d_keypoint/rtmpose-t_8xb256-120e_lapa-256x256.py) | 256x256 | 1.67 | 0.652 | - | - | [pth](https://download.openmmlab.com/mmpose/v1/projects/rtmposev1/rtmpose-t_simcc-face6_pt-in1k_120e-256x256-df79d9a5_20230529.pth)<br>[onnx](https://download.openmmlab.com/mmpose/v1/projects/rtmposev1/onnx_sdk/rtmpose-t_simcc-face6_pt-in1k_120e-256x256-df79d9a5_20230529.zip) |
|
||
| [RTMPose-s\*](./rtmpose/face_2d_keypoint/rtmpose-s_8xb256-120e_lapa-256x256.py) | 256x256 | 1.59 | 1.119 | - | - | [pth](https://download.openmmlab.com/mmpose/v1/projects/rtmposev1/rtmpose-s_simcc-face6_pt-in1k_120e-256x256-d779fdef_20230529.pth)<br>[onnx](https://download.openmmlab.com/mmpose/v1/projects/rtmposev1/onnx_sdk/rtmpose-s_simcc-face6_pt-in1k_120e-256x256-d779fdef_20230529.zip) |
|
||
| [RTMPose-m\*](./rtmpose/face_2d_keypoint/rtmpose-m_8xb256-120e_lapa-256x256.py) | 256x256 | 1.44 | 2.852 | - | - | [pth](https://download.openmmlab.com/mmpose/v1/projects/rtmposev1/rtmpose-m_simcc-face6_pt-in1k_120e-256x256-72a37400_20230529.pth)<br>[onnx](https://download.openmmlab.com/mmpose/v1/projects/rtmposev1/onnx_sdk/rtmpose-m_simcc-face6_pt-in1k_120e-256x256-72a37400_20230529.zip) |
|
||
|
||
</details>
|
||
|
||
### 手部 2d 关键点 (21 Keypoints)
|
||
|
||
- 关键点骨架定义遵循 [COCO-WholeBody](https://github.com/jin-s13/COCO-WholeBody/),详情见 [meta info](/configs/_base_/datasets/coco_wholebody_hand.py)。
|
||
- <img src="https://user-images.githubusercontent.com/100993824/227771101-03a27bd8-ccc0-4eb9-a111-660f191a7a16.png" height="300px">
|
||
|
||
| Detection Config | Input Size | Model AP<sup><br>(OneHand10K) | Flops<sup><br>(G) | ORT-Latency<sup><br>(ms)<sup><br>(i7-11700) | TRT-FP16-Latency<sup><br>(ms)<sup><br>(GTX 1660Ti) | Download |
|
||
| :---------------------------: | :--------: | :---------------------------: | :---------------: | :-----------------------------------------: | :------------------------------------------------: | :--------------------: |
|
||
| [RTMDet-nano<sup><br>(alpha version)](./rtmdet/hand/rtmdet_nano_320-8xb32_hand.py) | 320x320 | 76.0 | 0.31 | - | - | [pth](https://download.openmmlab.com/mmpose/v1/projects/rtmposev1/rtmdet_nano_8xb32-300e_hand-267f9c8f.pth)<br>[onnx](https://download.openmmlab.com/mmpose/v1/projects/rtmposev1/onnx_sdk/rtmdet_nano_8xb32-300e_hand-267f9c8f.zip) |
|
||
|
||
<details open>
|
||
<summary><b>Hand5</b></summary>
|
||
|
||
- `Hand5` and `*` 代表模型在 5 个开源数据集上训练得到:
|
||
- [COCO-Wholebody-Hand](https://github.com/jin-s13/COCO-WholeBody/)
|
||
- [OneHand10K](https://www.yangangwang.com/papers/WANG-MCC-2018-10.html)
|
||
- [FreiHand2d](https://lmb.informatik.uni-freiburg.de/projects/freihand/)
|
||
- [RHD2d](https://lmb.informatik.uni-freiburg.de/resources/datasets/RenderedHandposeDataset.en.html)
|
||
- [Halpe](https://mmpose.readthedocs.io/en/latest/dataset_zoo/2d_wholebody_keypoint.html#halpe)
|
||
|
||
| Config | Input Size | PCK@0.2<sup><br>(COCO-Wholebody-Hand) | PCK@0.2<sup><br>(Hand5) | AUC<sup><br>(Hand5) | FLOPS<sup><br>(G) | ORT-Latency<sup><br>(ms)<sup><br>(i7-11700) | TRT-FP16-Latency<sup><br>(ms)<sup><br>(GTX 1660Ti) | Download |
|
||
| :-------------------------------------------------------------------------------------------------------------------: | :--------: | :-----------------------------------: | :---------------------: | :-----------------: | :---------------: | :-----------------------------------------: | :------------------------------------------------: | :----------------------------------------------------------------------------------------------------------------------------------------------------------------------------------------------------------------------------------------------------------------------------------------: |
|
||
| [RTMPose-m\*<sup><br>(alpha version)](./rtmpose/hand_2d_keypoint/rtmpose-m_8xb32-210e_coco-wholebody-hand-256x256.py) | 256x256 | 81.5 | 96.4 | 83.9 | 2.581 | - | - | [pth](https://download.openmmlab.com/mmpose/v1/projects/rtmposev1/rtmpose-m_simcc-hand5_pt-aic-coco_210e-256x256-74fb594_20230320.pth)<br>[onnx](https://download.openmmlab.com/mmpose/v1/projects/rtmposev1/onnx_sdk/rtmpose-m_simcc-hand5_pt-aic-coco_210e-256x256-74fb594_20230320.zip) |
|
||
|
||
</details>
|
||
|
||
### 预训练模型
|
||
|
||
我们提供了 UDP 预训练的 CSPNeXt 模型参数,训练配置请参考 [pretrain_cspnext_udp folder](./rtmpose/pretrain_cspnext_udp/)。
|
||
|
||
<details close>
|
||
<summary><b>AIC+COCO</b></summary>
|
||
|
||
| Model | Input Size | Params<sup><br>(M) | Flops<sup><br>(G) | AP<sup><br>(GT) | AR<sup><br>(GT) | Download |
|
||
| :----------: | :--------: | :----------------: | :---------------: | :-------------: | :-------------: | :---------------------------------------------------------------------------------------------------------------: |
|
||
| CSPNeXt-tiny | 256x192 | 6.03 | 1.43 | 65.5 | 68.9 | [pth](https://download.openmmlab.com/mmpose/v1/projects/rtmpose/cspnext-tiny_udp-aic-coco_210e-256x192-cbed682d_20230130.pth) |
|
||
| CSPNeXt-s | 256x192 | 8.58 | 1.78 | 70.0 | 73.3 | [pth](https://download.openmmlab.com/mmpose/v1/projects/rtmpose/cspnext-s_udp-aic-coco_210e-256x192-92f5a029_20230130.pth) |
|
||
| CSPNeXt-m | 256x192 | 17.53 | 3.05 | 74.8 | 77.7 | [pth](https://download.openmmlab.com/mmpose/v1/projects/rtmpose/cspnext-m_udp-aic-coco_210e-256x192-f2f7d6f6_20230130.pth) |
|
||
| CSPNeXt-l | 256x192 | 32.44 | 5.32 | 77.2 | 79.9 | [pth](https://download.openmmlab.com/mmpose/v1/projects/rtmpose/cspnext-l_udp-aic-coco_210e-256x192-273b7631_20230130.pth) |
|
||
|
||
</details>
|
||
|
||
<details open>
|
||
<summary><b>Body8</b></summary>
|
||
|
||
- `*` 代表模型在 7 个开源数据集上训练得到:
|
||
- [AI Challenger](https://mmpose.readthedocs.io/en/latest/dataset_zoo/2d_body_keypoint.html#aic)
|
||
- [MS COCO](https://mmpose.readthedocs.io/en/latest/dataset_zoo/2d_body_keypoint.html#coco)
|
||
- [CrowdPose](https://mmpose.readthedocs.io/en/latest/dataset_zoo/2d_body_keypoint.html#crowdpose)
|
||
- [MPII](https://mmpose.readthedocs.io/en/latest/dataset_zoo/2d_body_keypoint.html#mpii)
|
||
- [sub-JHMDB](https://mmpose.readthedocs.io/en/latest/dataset_zoo/2d_body_keypoint.html#sub-jhmdb-dataset)
|
||
- [Halpe](https://mmpose.readthedocs.io/en/latest/dataset_zoo/2d_wholebody_keypoint.html#halpe)
|
||
- [PoseTrack18](https://mmpose.readthedocs.io/en/latest/dataset_zoo/2d_body_keypoint.html#posetrack18)
|
||
- `Body8` 代表除了以上提到的 7 个数据集,再加上 [OCHuman](https://mmpose.readthedocs.io/en/latest/dataset_zoo/2d_body_keypoint.html#ochuman) 合并后一起进行评测得到的指标。
|
||
|
||
| Model | Input Size | Params<sup><br>(M) | Flops<sup><br>(G) | AP<sup><br>(COCO) | PCK@0.2<sup><br>(Body8) | AUC<sup><br>(Body8) | Download |
|
||
| :------------: | :--------: | :----------------: | :---------------: | :---------------: | :---------------------: | :-----------------: | :--------------------------------------------------------------------------------: |
|
||
| CSPNeXt-tiny\* | 256x192 | 6.03 | 1.43 | 65.9 | 96.34 | 63.80 | [pth](https://download.openmmlab.com/mmpose/v1/projects/rtmposev1/cspnext-tiny_udp-body7_210e-256x192-a3775292_20230504.pth) |
|
||
| CSPNeXt-s\* | 256x192 | 8.58 | 1.78 | 68.7 | 96.59 | 64.92 | [pth](https://download.openmmlab.com/mmpose/v1/projects/rtmposev1/cspnext-s_udp-body7_210e-256x192-8c9ccbdb_20230504.pth) |
|
||
| CSPNeXt-m\* | 256x192 | 17.53 | 3.05 | 73.7 | 97.42 | 68.19 | [pth](https://download.openmmlab.com/mmpose/v1/projects/rtmposev1/cspnext-m_udp-body7_210e-256x192-e0c9327b_20230504.pth) |
|
||
| CSPNeXt-l\* | 256x192 | 32.44 | 5.32 | 75.7 | 97.76 | 69.57 | [pth](https://download.openmmlab.com/mmpose/v1/projects/rtmposev1/cspnext-l_udp-body7_210e-256x192-5e9558ef_20230504.pth) |
|
||
| CSPNeXt-m\* | 384x288 | 17.53 | 6.86 | 75.8 | 97.60 | 70.18 | [pth](https://download.openmmlab.com/mmpose/v1/projects/rtmposev1/cspnext-m_udp-body7_210e-384x288-b9bc2b57_20230504.pth) |
|
||
| CSPNeXt-l\* | 384x288 | 32.44 | 11.96 | 77.2 | 97.89 | 71.23 | [pth](https://download.openmmlab.com/mmpose/v1/projects/rtmposev1/cspnext-l_udp-body7_210e-384x288-b15bc30d_20230504.pth) |
|
||
| CSPNeXt-x\* | 384x288 | 54.92 | 19.96 | 78.1 | 98.00 | 71.79 | [pth](https://download.openmmlab.com/mmpose/v1/projects/rtmposev1/cspnext-x_udp-body7_210e-384x288-d28b58e6_20230529.pth) |
|
||
|
||
</details>
|
||
|
||
#### ImageNet
|
||
|
||
我们提供了 ImageNet 分类训练的 CSPNeXt 模型参数,更多细节请参考 [RTMDet](https://github.com/open-mmlab/mmdetection/blob/latest/configs/rtmdet/README.md#classification)。
|
||
|
||
| Model | Input Size | Params<sup><br>(M) | Flops<sup><br>(G) | Top-1 (%) | Top-5 (%) | Download |
|
||
| :----------: | :--------: | :----------------: | :---------------: | :-------: | :-------: | :---------------------------------------------------------------------------------------------------------------------------: |
|
||
| CSPNeXt-tiny | 224x224 | 2.73 | 0.34 | 69.44 | 89.45 | [pth](https://download.openmmlab.com/mmdetection/v3.0/rtmdet/cspnext_rsb_pretrain/cspnext-tiny_imagenet_600e-3a2dd350.pth) |
|
||
| CSPNeXt-s | 224x224 | 4.89 | 0.66 | 74.41 | 92.23 | [pth](https://download.openmmlab.com/mmdetection/v3.0/rtmdet/cspnext_rsb_pretrain/cspnext-s_imagenet_600e-ea671761.pth) |
|
||
| CSPNeXt-m | 224x224 | 13.05 | 1.93 | 79.27 | 94.79 | [pth](https://download.openmmlab.com/mmdetection/v3.0/rtmdet/cspnext_rsb_pretrain/cspnext-m_8xb256-rsb-a1-600e_in1k-ecb3bbd9.pth) |
|
||
| CSPNeXt-l | 224x224 | 27.16 | 4.19 | 81.30 | 95.62 | [pth](https://download.openmmlab.com/mmdetection/v3.0/rtmdet/cspnext_rsb_pretrain/cspnext-l_8xb256-rsb-a1-600e_in1k-6a760974.pth) |
|
||
| CSPNeXt-x | 224x224 | 48.85 | 7.76 | 82.10 | 95.69 | [pth](https://download.openmmlab.com/mmdetection/v3.0/rtmdet/cspnext_rsb_pretrain/cspnext-x_8xb256-rsb-a1-600e_in1k-b3f78edd.pth) |
|
||
|
||
## 👀 可视化 [🔝](#-table-of-contents)
|
||
|
||
<div align=center>
|
||
<img src='https://user-images.githubusercontent.com/13503330/221795678-2c4ae2ec-ac23-4368-8083-0ebeb29f0d3c.gif' width=900/>
|
||
<img src="https://user-images.githubusercontent.com/13503330/219270443-421d9b02-fcec-46de-90f2-ce769c67575a.png" width=900 />
|
||
</div>
|
||
|
||
## 😎 快速尝试 [🔝](#-table-of-contents)
|
||
|
||
我们提供了多种途径来让用户尝试 RTMPose 模型:
|
||
|
||
- [在线 RTMPose Demo](https://openxlab.org.cn/apps/detail/mmpose/RTMPose)
|
||
- [Examples](https://github.com/open-mmlab/mmpose/tree/dev-1.x/projects/rtmpose/examples/onnxruntime) 基于 Python 和 ONNXRuntime (无 MMCV 依赖)
|
||
- [rtmlib 推理库](https://github.com/Tau-J/rtmlib/tree/main) (无 MMCV、Pytorch 依赖)
|
||
- MMPose demo 脚本 (基于 Pytorch)
|
||
- MMDeploy SDK 预编译包 (推荐,速度提升6-10倍)
|
||
|
||
### rtmlib 推理库
|
||
|
||
[rtmlib](https://github.com/Tau-J/rtmlib/tree/main) 提供了开箱即用的 RTMPose 全系列官方及衍生模型的推理:
|
||
|
||
- 无需安装 mmcv,mmengine,mmpose 等一系列训练库,无需 pytorch 环境,有 opencv 就能推理
|
||
- 超级友好简洁的推理和可视化接口
|
||
- 支持 CPU 和 GPU 推理
|
||
- 自动下载和缓存 onnx 模型
|
||
- 支持 RTMPose 全系列官方及衍生模型:RTMPose,DWPose,RTMO,RTMW etc.
|
||
|
||
### MMPose demo 脚本
|
||
|
||
通过 MMPose 提供的 demo 脚本可以基于 Pytorch 快速进行[模型推理](https://mmpose.readthedocs.io/en/latest/user_guides/inference.html)和效果验证。
|
||
|
||
**提示:**
|
||
|
||
- 基于 Pytorch 推理并不能达到 RTMPose 模型的最大推理速度,只用于模型效果验证。
|
||
- 输入模型路径可以是本地路径,也可以是下载链接。
|
||
|
||
```shell
|
||
# 前往 mmpose 目录
|
||
cd ${PATH_TO_MMPOSE}
|
||
|
||
# RTMDet 与 RTMPose 联合推理
|
||
# 输入模型路径可以是本地路径,也可以是下载链接。
|
||
python demo/topdown_demo_with_mmdet.py \
|
||
projects/rtmpose/rtmdet/person/rtmdet_nano_320-8xb32_coco-person.py \
|
||
https://download.openmmlab.com/mmpose/v1/projects/rtmpose/rtmdet_nano_8xb32-100e_coco-obj365-person-05d8511e.pth \
|
||
projects/rtmpose/rtmpose/body_2d_keypoint/rtmpose-m_8xb256-420e_coco-256x192.py \
|
||
https://download.openmmlab.com/mmpose/v1/projects/rtmposev1/rtmpose-m_simcc-aic-coco_pt-aic-coco_420e-256x192-63eb25f7_20230126.pth \
|
||
--input {YOUR_TEST_IMG_or_VIDEO} \
|
||
--show
|
||
|
||
# 摄像头推理
|
||
# 输入模型路径可以是本地路径,也可以是下载链接。
|
||
python demo/topdown_demo_with_mmdet.py \
|
||
projects/rtmpose/rtmdet/person/rtmdet_nano_320-8xb32_coco-person.py \
|
||
https://download.openmmlab.com/mmpose/v1/projects/rtmpose/rtmdet_nano_8xb32-100e_coco-obj365-person-05d8511e.pth \
|
||
projects/rtmpose/rtmpose/body_2d_keypoint/rtmpose-m_8xb256-420e_coco-256x192.py \
|
||
https://download.openmmlab.com/mmpose/v1/projects/rtmposev1/rtmpose-m_simcc-aic-coco_pt-aic-coco_420e-256x192-63eb25f7_20230126.pth \
|
||
--input webcam \
|
||
--show
|
||
```
|
||
|
||
效果展示:
|
||
|
||
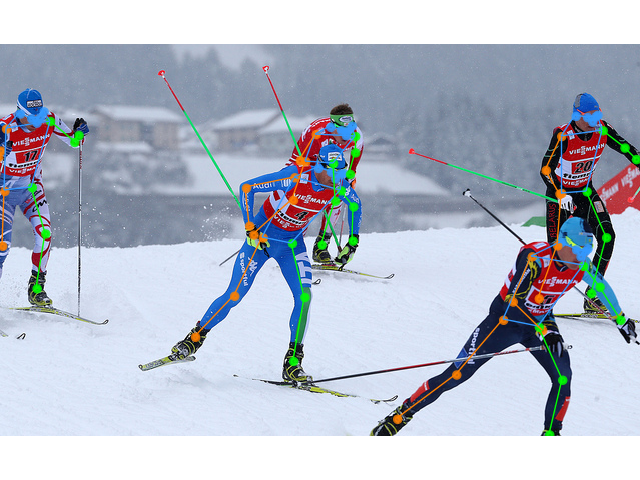
|
||
|
||
### MMDeploy SDK 预编译包 (推荐)
|
||
|
||
MMDeploy 提供了预编译的 SDK,用于对 RTMPose 项目进行 Pipeline 推理,其中推理所用的模型为 SDK 版本。
|
||
|
||
- 所有的模型必须经过 `tools/deploy.py` 导出后才能使用 PoseTracker 进行推理。
|
||
- 导出 SDK 版模型的教程见 [SDK 推理](#%EF%B8%8F-sdk-推理),推理的详细参数设置见 [Pipeline 推理](#-pipeline-推理)。
|
||
- 你可以从 [硬件模型库](https://platform.openmmlab.com/deploee) 直接下载 SDK 版模型(ONNX、 TRT、ncnn 等)。
|
||
- 同时我们也支持 [在线模型转换](https://platform.openmmlab.com/deploee/task-convert-list)。
|
||
|
||
#### Linux\\
|
||
|
||
说明:
|
||
|
||
- GCC 版本需大于 7.5
|
||
- cmake 版本需大于 3.20
|
||
|
||
##### Python 推理
|
||
|
||
1. 安装 mmdeploy_runtime 或者 mmdeploy_runtime_gpu
|
||
|
||
```shell
|
||
# for onnxruntime
|
||
pip install mmdeploy-runtime
|
||
|
||
# for onnxruntime-gpu / tensorrt
|
||
pip install mmdeploy-runtime-gpu
|
||
```
|
||
|
||
2. 下载预编译包:
|
||
|
||
```shell
|
||
# onnxruntime
|
||
# for ubuntu
|
||
wget -c https://github.com/open-mmlab/mmdeploy/releases/download/v1.0.0/mmdeploy-1.0.0-linux-x86_64-cxx11abi.tar.gz
|
||
# 解压并将 third_party 中第三方推理库的动态库添加到 PATH
|
||
|
||
# for centos7 and lower
|
||
wget -c https://github.com/open-mmlab/mmdeploy/releases/download/v1.0.0/mmdeploy-1.0.0-linux-x86_64.tar.gz
|
||
# 解压并将 third_party 中第三方推理库的动态库添加到 PATH
|
||
|
||
# onnxruntime-gpu / tensorrt
|
||
# for ubuntu
|
||
wget -c https://github.com/open-mmlab/mmdeploy/releases/download/v1.0.0/mmdeploy-1.0.0-linux-x86_64-cxx11abi-cuda11.3.tar.gz
|
||
# 解压并将 third_party 中第三方推理库的动态库添加到 PATH
|
||
|
||
# for centos7 and lower
|
||
wget -c https://github.com/open-mmlab/mmdeploy/releases/download/v1.0.0/mmdeploy-1.0.0-linux-x86_64-cuda11.3.tar.gz
|
||
# 解压并将 third_party 中第三方推理库的动态库添加到 PATH
|
||
```
|
||
|
||
3. 下载 sdk 模型并解压到 `./example/python` 下。(该模型只用于演示,如需其他模型,请参考 [SDK 推理](#%EF%B8%8F-sdk-推理))
|
||
|
||
```shell
|
||
# rtmdet-nano + rtmpose-m for cpu sdk
|
||
wget https://download.openmmlab.com/mmpose/v1/projects/rtmpose/rtmpose-cpu.zip
|
||
|
||
unzip rtmpose-cpu.zip
|
||
```
|
||
|
||
4. 使用 `pose_tracker.py` 进行推理:
|
||
|
||
```shell
|
||
# 进入 ./example/python
|
||
|
||
# 请传入模型目录,而不是模型文件
|
||
# 格式:
|
||
# python pose_tracker.py cpu {det work-dir} {pose work-dir} {your_video.mp4}
|
||
|
||
# 示例:
|
||
python pose_tracker.py cpu rtmpose-ort/rtmdet-nano/ rtmpose-ort/rtmpose-m/ your_video.mp4
|
||
|
||
# 摄像头
|
||
python pose_tracker.py cpu rtmpose-ort/rtmdet-nano/ rtmpose-ort/rtmpose-m/ 0
|
||
```
|
||
|
||
##### ONNX
|
||
|
||
```shell
|
||
# 下载预编译包
|
||
wget https://github.com/open-mmlab/mmdeploy/releases/download/v1.0.0/mmdeploy-1.0.0-linux-x86_64-cxx11abi.tar.gz
|
||
|
||
# 解压文件
|
||
tar -xzvf mmdeploy-1.0.0-linux-x86_64-cxx11abi.tar.gz
|
||
|
||
# 切换到 sdk 目录
|
||
cd mmdeploy-1.0.0-linux-x86_64-cxx11abi
|
||
|
||
# 设置环境变量
|
||
source set_env.sh
|
||
|
||
# 如果系统中没有安装 opencv 3+,请执行以下命令。如果已安装,可略过
|
||
bash install_opencv.sh
|
||
|
||
# 编译可执行程序
|
||
bash build_sdk.sh
|
||
|
||
# 图片推理
|
||
# 请传入模型目录,而不是模型文件
|
||
./bin/det_pose rtmpose-ort/rtmdet-nano/ rtmpose-ort/rtmpose-m/ your_img.jpg --device cpu
|
||
|
||
# 视频推理
|
||
# 请传入模型目录,而不是模型文件
|
||
./bin/pose_tracker rtmpose-ort/rtmdet-nano/ rtmpose-ort/rtmpose-m/ your_video.mp4 --device cpu
|
||
|
||
# 摄像头推理
|
||
# 请传入模型目录,而不是模型文件
|
||
./bin/pose_tracker rtmpose-ort/rtmdet-nano/ rtmpose-ort/rtmpose-m/ 0 --device cpu
|
||
```
|
||
|
||
##### TensorRT
|
||
|
||
```shell
|
||
# 下载预编译包
|
||
wget https://github.com/open-mmlab/mmdeploy/releases/download/v1.0.0/mmdeploy-1.0.0-linux-x86_64-cxx11abi-cuda11.3.tar.gz
|
||
|
||
# 解压文件
|
||
tar -xzvf mmdeploy-1.0.0-linux-x86_64-cxx11abi-cuda11.3.tar.gz
|
||
|
||
# 切换到 sdk 目录
|
||
cd mmdeploy-1.0.0-linux-x86_64-cxx11abi-cuda11.3
|
||
|
||
# 设置环境变量
|
||
source set_env.sh
|
||
|
||
# 如果系统中没有安装 opencv 3+,请执行以下命令。如果已安装,可略过
|
||
bash install_opencv.sh
|
||
|
||
# 编译可执行程序
|
||
bash build_sdk.sh
|
||
|
||
# 图片推理
|
||
# 请传入模型目录,而不是模型文件
|
||
./bin/det_pose rtmpose-ort/rtmdet-nano/ rtmpose-ort/rtmpose-m/ your_img.jpg --device cuda
|
||
|
||
# 视频推理
|
||
# 请传入模型目录,而不是模型文件
|
||
./bin/pose_tracker rtmpose-ort/rtmdet-nano/ rtmpose-ort/rtmpose-m/ your_video.mp4 --device cuda
|
||
|
||
# 摄像头推理
|
||
# 请传入模型目录,而不是模型文件
|
||
./bin/pose_tracker rtmpose-ort/rtmdet-nano/ rtmpose-ort/rtmpose-m/ 0 --device cuda
|
||
```
|
||
|
||
详细参数设置见 [Pipeline 推理](#-pipeline-推理)。
|
||
|
||
#### Windows
|
||
|
||
##### Python 推理
|
||
|
||
1. 安装 mmdeploy_runtime 或者 mmdeploy_runtime_gpu
|
||
|
||
```shell
|
||
# for onnxruntime
|
||
pip install mmdeploy-runtime
|
||
# 下载 [sdk](https://github.com/open-mmlab/mmdeploy/releases/download/v1.0.0/mmdeploy-1.0.0-windows-amd64.zip) 并将 third_party 中第三方推理库的动态库添加到 PATH
|
||
|
||
# for onnxruntime-gpu / tensorrt
|
||
pip install mmdeploy-runtime-gpu
|
||
# 下载 [sdk](https://github.com/open-mmlab/mmdeploy/releases/download/v1.0.0/mmdeploy-1.0.0-windows-amd64-cuda11.3.zip) 并将 third_party 中第三方推理库的动态库添加到 PATH
|
||
```
|
||
|
||
2. 下载 sdk 模型并解压到 `./example/python` 下。(该模型只用于演示,如需其他模型,请参考 [SDK 推理](#%EF%B8%8F-sdk-推理))
|
||
|
||
```shell
|
||
# rtmdet-nano + rtmpose-m for cpu sdk
|
||
wget https://download.openmmlab.com/mmpose/v1/projects/rtmpose/rtmpose-cpu.zip
|
||
|
||
unzip rtmpose-cpu.zip
|
||
```
|
||
|
||
3. 使用 `pose_tracker.py` 进行推理:
|
||
|
||
```shell
|
||
# 进入 ./example/python
|
||
# 请传入模型目录,而不是模型文件
|
||
python pose_tracker.py cpu rtmpose-ort/rtmdet-nano/ rtmpose-ort/rtmpose-m/ your_video.mp4
|
||
|
||
# 摄像头
|
||
# 请传入模型目录,而不是模型文件
|
||
python pose_tracker.py cpu rtmpose-ort/rtmdet-nano/ rtmpose-ort/rtmpose-m/ 0
|
||
```
|
||
|
||
##### 可执行文件推理
|
||
|
||
1. 安装 [cmake](https://cmake.org/download/)。
|
||
2. 前往 [mmdeploy](https://github.com/open-mmlab/mmdeploy/releases) 下载 win 预编译包。
|
||
3. 解压文件,进入 sdk 目录。
|
||
4. 使用管理员权限打开 PowerShell,执行以下命令:
|
||
|
||
```shell
|
||
set-ExecutionPolicy RemoteSigned
|
||
```
|
||
|
||
5. 安装 OpenCV:
|
||
|
||
```shell
|
||
# in sdk folder:
|
||
.\install_opencv.ps1
|
||
```
|
||
|
||
6. 配置环境变量:
|
||
|
||
```shell
|
||
# in sdk folder:
|
||
. .\set_env.ps1
|
||
```
|
||
|
||
7. 编译 sdk:
|
||
|
||
```shell
|
||
# in sdk folder:
|
||
# 如果你通过 .\install_opencv.ps1 安装 opencv,直接运行如下指令:
|
||
.\build_sdk.ps1
|
||
# 如果你自行安装了 opencv,需要指定 OpenCVConfig.cmake 的路径:
|
||
.\build_sdk.ps1 "path/to/folder/of/OpenCVConfig.cmake"
|
||
```
|
||
|
||
8. 可执行文件会在如下路径生成:
|
||
|
||
```shell
|
||
example\cpp\build\Release
|
||
```
|
||
|
||
### MMPose demo 脚本
|
||
|
||
通过 MMPose 提供的 demo 脚本可以基于 Pytorch 快速进行[模型推理](https://mmpose.readthedocs.io/en/latest/user_guides/inference.html)和效果验证。
|
||
|
||
**提示:**
|
||
|
||
- 基于 Pytorch 推理并不能达到 RTMPose 模型的真实推理速度,只用于模型效果验证。
|
||
|
||
```shell
|
||
# 前往 mmpose 目录
|
||
cd ${PATH_TO_MMPOSE}
|
||
|
||
# RTMDet 与 RTMPose 联合推理
|
||
python demo/topdown_demo_with_mmdet.py \
|
||
projects/rtmpose/rtmdet/person/rtmdet_nano_320-8xb32_coco-person.py \
|
||
{PATH_TO_CHECKPOINT}/rtmdet_nano_8xb32-100e_coco-obj365-person-05d8511e.pth \
|
||
projects/rtmpose/rtmpose/body_2d_keypoint/rtmpose-m_8xb256-420e_coco-256x192.py \
|
||
{PATH_TO_CHECKPOINT}/rtmpose-m_simcc-aic-coco_pt-aic-coco_420e-256x192-63eb25f7_20230126.pth \
|
||
--input {YOUR_TEST_IMG_or_VIDEO} \
|
||
--show
|
||
|
||
# 摄像头推理
|
||
python demo/topdown_demo_with_mmdet.py \
|
||
projects/rtmpose/rtmdet/person/rtmdet_nano_320-8xb32_coco-person.py \
|
||
{PATH_TO_CHECKPOINT}/rtmdet_nano_8xb32-100e_coco-obj365-person-05d8511e.pth \
|
||
projects/rtmpose/rtmpose/body_2d_keypoint/rtmpose-m_8xb256-420e_coco-256x192.py \
|
||
{PATH_TO_CHECKPOINT}/rtmpose-m_simcc-aic-coco_pt-aic-coco_420e-256x192-63eb25f7_20230126.pth \
|
||
--input webcam \
|
||
--show
|
||
```
|
||
|
||
效果展示:
|
||
|
||
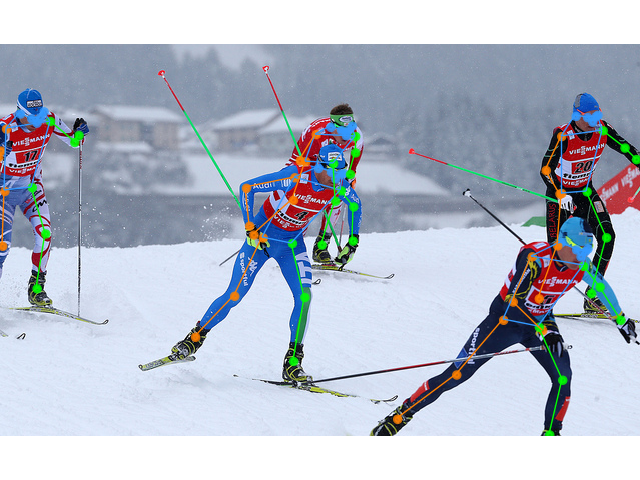
|
||
|
||
## 👨🏫 模型训练 [🔝](#-table-of-contents)
|
||
|
||
请参考 [训练与测试](https://mmpose.readthedocs.io/en/latest/user_guides/train_and_test.html) 进行 RTMPose 的训练。
|
||
|
||
**提示**:
|
||
|
||
- 当用户的数据集较小时请根据情况缩小 `batch_size` 和 `base_lr`。
|
||
- 模型选择
|
||
- m:推荐首选使用
|
||
- t/s:适用于极端低算力的移动设备,或对推理速度要求严格的场景
|
||
- l:适用于算力较强、对速度不敏感的场景
|
||
|
||
## 🏗️ 部署教程 [🔝](#-table-of-contents)
|
||
|
||
本教程将展示如何通过 [MMDeploy](https://github.com/open-mmlab/mmdeploy/tree/main) 部署 RTMPose 项目。
|
||
|
||
- 你可以从 [硬件模型库](https://platform.openmmlab.com/deploee) 直接下载 SDK 版模型(ONNX、 TRT、ncnn 等)。
|
||
- 同时我们也支持 [在线模型转换](https://platform.openmmlab.com/deploee/task-convert-list)。
|
||
|
||
### 🧩 安装
|
||
|
||
在开始部署之前,首先你需要确保正确安装了 MMPose, MMDetection, MMDeploy,相关安装教程如下:
|
||
|
||
- [安装 MMPose 与 MMDetection](https://mmpose.readthedocs.io/zh_CN/latest/installation.html)
|
||
- [安装 MMDeploy](https://mmdeploy.readthedocs.io/zh_CN/latest/04-supported-codebases/mmpose.html)
|
||
|
||
根据部署后端的不同,有的后端需要对自定义算子进行编译,请根据需求前往对应的文档确保环境搭建正确:
|
||
|
||
- [ONNX](https://mmdeploy.readthedocs.io/zh_CN/latest/05-supported-backends/onnxruntime.html)
|
||
- [TensorRT](https://mmdeploy.readthedocs.io/zh_CN/latest/05-supported-backends/tensorrt.html)
|
||
- [OpenVINO](https://mmdeploy.readthedocs.io/zh_CN/latest/05-supported-backends/openvino.html)
|
||
- [TorchScript](https://mmdeploy.readthedocs.io/en/latest/05-supported-backends/torchscript.html)
|
||
- [更多](https://github.com/open-mmlab/mmdeploy/tree/main/docs/en/05-supported-backends)
|
||
|
||
### 🛠️ 模型转换
|
||
|
||
在完成安装之后,你就可以开始模型部署了。通过 MMDeploy 提供的 `tools/deploy.py` 可以方便地将 Pytorch 模型转换到不同的部署后端。
|
||
|
||
我们本节演示将 RTMDet 和 RTMPose 模型导出为 ONNX 和 TensorRT 格式,如果你希望了解更多内容请前往 [MMDeploy 文档](https://mmdeploy.readthedocs.io/zh_CN/latest/02-how-to-run/convert_model.html)。
|
||
|
||
- ONNX 配置
|
||
|
||
\- RTMDet:[`detection_onnxruntime_static.py`](https://github.com/open-mmlab/mmdeploy/blob/main/configs/mmdet/detection/detection_onnxruntime_static.py)
|
||
|
||
\- RTMPose:[`pose-detection_simcc_onnxruntime_dynamic.py`](https://github.com/open-mmlab/mmdeploy/blob/main/configs/mmpose/pose-detection_simcc_onnxruntime_dynamic.py)
|
||
|
||
- TensorRT 配置
|
||
|
||
\- RTMDet:[`detection_tensorrt_static-320x320.py`](https://github.com/open-mmlab/mmdeploy/blob/main/configs/mmdet/detection/detection_tensorrt_static-320x320.py)
|
||
|
||
\- RTMPose:[`pose-detection_simcc_tensorrt_dynamic-256x192.py`](https://github.com/open-mmlab/mmdeploy/blob/main/configs/mmpose/pose-detection_simcc_tensorrt_dynamic-256x192.py)
|
||
|
||
- 更多
|
||
|
||
| Backend | Config |
|
||
| :---------: | :------------------------------------------------------------------------------------------------------------------------------------------------------------------: |
|
||
| ncnn-fp16 | [pose-detection_simcc_ncnn-fp16_static-256x192.py](https://github.com/open-mmlab/mmdeploy/blob/main/configs/mmpose/pose-detection_simcc_ncnn-fp16_static-256x192.py) |
|
||
| CoreML | [pose-detection_simcc_coreml_static-256x192.py](https://github.com/open-mmlab/mmdeploy/blob/main/configs/mmpose/pose-detection_simcc_coreml_static-256x192.py) |
|
||
| OpenVINO | [pose-detection_simcc_openvino_static-256x192.py](https://github.com/open-mmlab/mmdeploy/blob/main/configs/mmpose/pose-detection_simcc_openvino_static-256x192.py) |
|
||
| RKNN | [pose-detection_simcc_rknn-fp16_static-256x192.py](https://github.com/open-mmlab/mmdeploy/blob/main/configs/mmpose/pose-detection_simcc_rknn-fp16_static-256x192.py) |
|
||
| TorchScript | [pose-detection_torchscript.py](https://github.com/open-mmlab/mmdeploy/blob/main/configs/mmpose/pose-detection_torchscript.py) |
|
||
|
||
如果你需要对部署配置进行修改,请参考 [MMDeploy config tutorial](https://mmdeploy.readthedocs.io/zh_CN/latest/02-how-to-run/write_config.html).
|
||
|
||
本教程中使用的文件结构如下:
|
||
|
||
```shell
|
||
|----mmdeploy
|
||
|----mmdetection
|
||
|----mmpose
|
||
```
|
||
|
||
#### ONNX
|
||
|
||
运行如下命令:
|
||
|
||
```shell
|
||
# 前往 mmdeploy 目录
|
||
cd ${PATH_TO_MMDEPLOY}
|
||
|
||
# 转换 RTMDet
|
||
# 输入模型路径可以是本地路径,也可以是下载链接。
|
||
python tools/deploy.py \
|
||
configs/mmdet/detection/detection_onnxruntime_static.py \
|
||
../mmpose/projects/rtmpose/rtmdet/person/rtmdet_nano_320-8xb32_coco-person.py \
|
||
https://download.openmmlab.com/mmpose/v1/projects/rtmpose/rtmdet_nano_8xb32-100e_coco-obj365-person-05d8511e.pth \
|
||
demo/resources/human-pose.jpg \
|
||
--work-dir rtmpose-ort/rtmdet-nano \
|
||
--device cpu \
|
||
--show \
|
||
--dump-info # 导出 sdk info
|
||
|
||
# 转换 RTMPose
|
||
# 输入模型路径可以是本地路径,也可以是下载链接。
|
||
python tools/deploy.py \
|
||
configs/mmpose/pose-detection_simcc_onnxruntime_dynamic.py \
|
||
../mmpose/projects/rtmpose/rtmpose/body_2d_keypoint/rtmpose-m_8xb256-420e_coco-256x192.py \
|
||
https://download.openmmlab.com/mmpose/v1/projects/rtmposev1/rtmpose-m_simcc-aic-coco_pt-aic-coco_420e-256x192-63eb25f7_20230126.pth \
|
||
demo/resources/human-pose.jpg \
|
||
--work-dir rtmpose-ort/rtmpose-m \
|
||
--device cpu \
|
||
--show \
|
||
--dump-info # 导出 sdk info
|
||
```
|
||
|
||
默认导出模型文件为 `{work-dir}/end2end.onnx`
|
||
|
||
#### TensorRT
|
||
|
||
运行如下命令:
|
||
|
||
```shell
|
||
# 前往 mmdeploy 目录
|
||
cd ${PATH_TO_MMDEPLOY}
|
||
|
||
# 转换 RTMDet
|
||
# 输入模型路径可以是本地路径,也可以是下载链接。
|
||
python tools/deploy.py \
|
||
configs/mmdet/detection/detection_tensorrt_static-320x320.py \
|
||
../mmpose/projects/rtmpose/rtmdet/person/rtmdet_nano_320-8xb32_coco-person.py \
|
||
https://download.openmmlab.com/mmpose/v1/projects/rtmpose/rtmdet_nano_8xb32-100e_coco-obj365-person-05d8511e.pth \
|
||
demo/resources/human-pose.jpg \
|
||
--work-dir rtmpose-trt/rtmdet-nano \
|
||
--device cuda:0 \
|
||
--show \
|
||
--dump-info # 导出 sdk info
|
||
|
||
# 转换 RTMPose
|
||
# 输入模型路径可以是本地路径,也可以是下载链接。
|
||
python tools/deploy.py \
|
||
configs/mmpose/pose-detection_simcc_tensorrt_dynamic-256x192.py \
|
||
../mmpose/projects/rtmpose/rtmpose/body_2d_keypoint/rtmpose-m_8xb256-420e_coco-256x192.py \
|
||
https://download.openmmlab.com/mmpose/v1/projects/rtmposev1/rtmpose-m_simcc-aic-coco_pt-aic-coco_420e-256x192-63eb25f7_20230126.pth \
|
||
demo/resources/human-pose.jpg \
|
||
--work-dir rtmpose-trt/rtmpose-m \
|
||
--device cuda:0 \
|
||
--show \
|
||
--dump-info # 导出 sdk info
|
||
```
|
||
|
||
默认导出模型文件为 `{work-dir}/end2end.engine`
|
||
|
||
🎊 如果模型顺利导出,你将会看到样例图片上的检测结果:
|
||
|
||
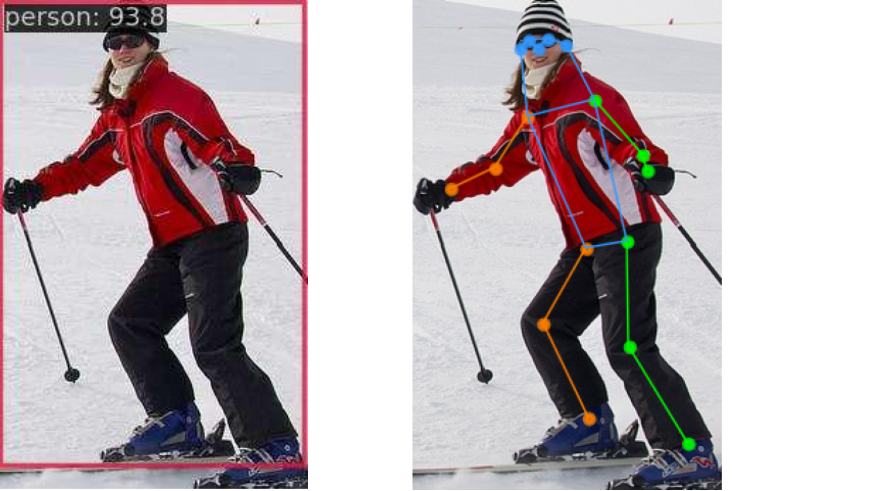
|
||
|
||
#### 高级设置
|
||
|
||
如果需要使用 TensorRT-FP16,你可以通过修改以下配置开启:
|
||
|
||
```Python
|
||
# in MMDeploy config
|
||
backend_config = dict(
|
||
type='tensorrt',
|
||
common_config=dict(
|
||
fp16_mode=True # 打开 fp16
|
||
))
|
||
```
|
||
|
||
### 🕹️ SDK 推理
|
||
|
||
要进行 Pipeline 推理,需要先用 MMDeploy 导出 SDK 版本的 det 和 pose 模型,只需要在参数中加上`--dump-info`。
|
||
|
||
此处以 onnxruntime 的 cpu 模型为例,运行如下命令:
|
||
|
||
```shell
|
||
# RTMDet
|
||
# 输入模型路径可以是本地路径,也可以是下载链接。
|
||
python tools/deploy.py \
|
||
configs/mmdet/detection/detection_onnxruntime_dynamic.py \
|
||
../mmpose/projects/rtmpose/rtmdet/person/rtmdet_nano_320-8xb32_coco-person.py \
|
||
https://download.openmmlab.com/mmpose/v1/projects/rtmpose/rtmdet_nano_8xb32-100e_coco-obj365-person-05d8511e.pth \
|
||
demo/resources/human-pose.jpg \
|
||
--work-dir rtmpose-ort/rtmdet-nano \
|
||
--device cpu \
|
||
--show \
|
||
--dump-info # 导出 sdk info
|
||
|
||
# RTMPose
|
||
# 输入模型路径可以是本地路径,也可以是下载链接。
|
||
python tools/deploy.py \
|
||
configs/mmpose/pose-detection_simcc_onnxruntime_dynamic.py \
|
||
../mmpose/projects/rtmpose/rtmpose/body_2d_keypoint/rtmpose-m_8xb256-420e_coco-256x192.py \
|
||
https://download.openmmlab.com/mmpose/v1/projects/rtmposev1/rtmpose-m_simcc-aic-coco_pt-aic-coco_420e-256x192-63eb25f7_20230126.pth \
|
||
demo/resources/human-pose.jpg \
|
||
--work-dir rtmpose-ort/rtmpose-m \
|
||
--device cpu \
|
||
--show \
|
||
--dump-info # 导出 sdk info
|
||
```
|
||
|
||
默认会导出三个 json 文件:
|
||
|
||
```shell
|
||
|----sdk
|
||
|----end2end.onnx # ONNX model
|
||
|----end2end.engine # TensorRT engine file
|
||
|
||
|----pipeline.json #
|
||
|----deploy.json # json files for the SDK
|
||
|----detail.json #
|
||
```
|
||
|
||
#### Python API
|
||
|
||
```Python
|
||
# Copyright (c) OpenMMLab. All rights reserved.
|
||
import argparse
|
||
|
||
import cv2
|
||
import numpy as np
|
||
from mmdeploy_runtime import PoseDetector
|
||
|
||
def parse_args():
|
||
parser = argparse.ArgumentParser(
|
||
description='show how to use sdk python api')
|
||
parser.add_argument('device_name', help='name of device, cuda or cpu')
|
||
parser.add_argument(
|
||
'model_path',
|
||
help='path of mmdeploy SDK model dumped by model converter')
|
||
parser.add_argument('image_path', help='path of an image')
|
||
parser.add_argument(
|
||
'--bbox',
|
||
default=None,
|
||
nargs='+',
|
||
type=int,
|
||
help='bounding box of an object in format (x, y, w, h)')
|
||
args = parser.parse_args()
|
||
return args
|
||
|
||
def main():
|
||
args = parse_args()
|
||
|
||
img = cv2.imread(args.image_path)
|
||
|
||
detector = PoseDetector(
|
||
model_path=args.model_path, device_name=args.device_name, device_id=0)
|
||
|
||
if args.bbox is None:
|
||
result = detector(img)
|
||
else:
|
||
# converter (x, y, w, h) -> (left, top, right, bottom)
|
||
print(args.bbox)
|
||
bbox = np.array(args.bbox, dtype=int)
|
||
bbox[2:] += bbox[:2]
|
||
result = detector(img, bbox)
|
||
print(result)
|
||
|
||
_, point_num, _ = result.shape
|
||
points = result[:, :, :2].reshape(point_num, 2)
|
||
for [x, y] in points.astype(int):
|
||
cv2.circle(img, (x, y), 1, (0, 255, 0), 2)
|
||
|
||
cv2.imwrite('output_pose.png', img)
|
||
|
||
if __name__ == '__main__':
|
||
main()
|
||
```
|
||
|
||
#### C++ API
|
||
|
||
```C++
|
||
#include "mmdeploy/pose_detector.hpp"
|
||
#include "opencv2/imgcodecs.hpp"
|
||
#include "opencv2/imgproc.hpp"
|
||
#include "utils/argparse.h" // See: https://github.com/open-mmlab/mmdeploy/blob/main/demo/csrc/cpp/utils/argparse.h
|
||
|
||
DEFINE_ARG_string(model_path, "Model path");
|
||
DEFINE_ARG_string(image_path, "Input image path");
|
||
DEFINE_string(device_name, "cpu", R"(Device name, e.g. "cpu", "cuda")");
|
||
DEFINE_int32(bbox_x, -1, R"(x position of the bounding box)");
|
||
DEFINE_int32(bbox_y, -1, R"(y position of the bounding box)");
|
||
DEFINE_int32(bbox_w, -1, R"(width of the bounding box)");
|
||
DEFINE_int32(bbox_h, -1, R"(height of the bounding box)");
|
||
|
||
int main(int argc, char* argv[]) {
|
||
if (!utils::ParseArguments(argc, argv)) {
|
||
return -1;
|
||
}
|
||
|
||
cv::Mat img = cv::imread(ARGS_image_path);
|
||
|
||
mmdeploy::PoseDetector detector(mmdeploy::Model{ARGS_model_path}, mmdeploy::Device{FLAGS_device_name, 0});
|
||
|
||
mmdeploy::PoseDetector::Result result{0, 0, nullptr};
|
||
|
||
if (FLAGS_bbox_x == -1 || FLAGS_bbox_y == -1 || FLAGS_bbox_w == -1 || FLAGS_bbox_h == -1) {
|
||
result = detector.Apply(img);
|
||
} else {
|
||
// convert (x, y, w, h) -> (left, top, right, bottom)
|
||
mmdeploy::cxx::Rect rect;
|
||
rect.left = FLAGS_bbox_x;
|
||
rect.top = FLAGS_bbox_y;
|
||
rect.right = FLAGS_bbox_x + FLAGS_bbox_w;
|
||
rect.bottom = FLAGS_bbox_y + FLAGS_bbox_h;
|
||
result = detector.Apply(img, {rect});
|
||
}
|
||
|
||
// Draw circles at detected keypoints
|
||
for (size_t i = 0; i < result[0].length; ++i) {
|
||
cv::Point keypoint(result[0].point[i].x, result[0].point[i].y);
|
||
cv::circle(img, keypoint, 1, cv::Scalar(0, 255, 0), 2); // Draw filled circle
|
||
}
|
||
|
||
// Save the output image
|
||
cv::imwrite("output_pose.png", img);
|
||
|
||
return 0;
|
||
}
|
||
```
|
||
|
||
对于 C++ API 示例,请将 MMDeploy 加入到 CMake 项目中:
|
||
|
||
```CMake
|
||
find_package(MMDeploy REQUIRED)
|
||
target_link_libraries(${name} PRIVATE mmdeploy ${OpenCV_LIBS})
|
||
```
|
||
|
||
#### 其他语言
|
||
|
||
- [C# API 示例](https://github.com/open-mmlab/mmdeploy/tree/main/demo/csharp)
|
||
- [JAVA API 示例](https://github.com/open-mmlab/mmdeploy/tree/main/demo/java)
|
||
|
||
### 🚀 Pipeline 推理
|
||
|
||
#### 图片推理
|
||
|
||
如果用户有跟随 MMDeploy 安装教程进行正确编译,在 `mmdeploy/build/bin/` 路径下会看到 `det_pose` 的可执行文件。
|
||
|
||
```shell
|
||
# 前往 mmdeploy 目录
|
||
cd ${PATH_TO_MMDEPLOY}/build/bin/
|
||
|
||
# 单张图片推理
|
||
./det_pose rtmpose-ort/rtmdet-nano/ rtmpose-ort/rtmpose-m/ your_img.jpg --device cpu
|
||
|
||
required arguments:
|
||
det_model Detection 模型路径 [string]
|
||
pose_model Pose 模型路径 [string]
|
||
image 输入图片路径 [string]
|
||
|
||
optional arguments:
|
||
--device 推理设备 "cpu", "cuda" [string = "cpu"]
|
||
--output 导出图片路径 [string = "det_pose_output.jpg"]
|
||
--skeleton 骨架定义文件路径,或使用预定义骨架:
|
||
"coco" [string = "coco", "coco-wholoebody"]
|
||
--det_label 用于姿势估计的检测标签 [int32 = 0]
|
||
(0 在 coco 中对应 person)
|
||
--det_thr 检测分数阈值 [double = 0.5]
|
||
--det_min_bbox_size 最小检测框大小 [double = -1]
|
||
--pose_thr 关键点置信度阈值 [double = 0]
|
||
```
|
||
|
||
**API** **示例**
|
||
|
||
\- [`det_pose.py`](https://github.com/open-mmlab/mmdeploy/blob/main/demo/python/det_pose.py)
|
||
|
||
\- [`det_pose.cxx`](https://github.com/open-mmlab/mmdeploy/blob/main/demo/csrc/cpp/det_pose.cxx)
|
||
|
||
#### 视频推理
|
||
|
||
如果用户有跟随 MMDeploy 安装教程进行正确编译,在 `mmdeploy/build/bin/` 路径下会看到 `pose_tracker` 的可执行文件。
|
||
|
||
- 将 `input` 输入 `0` 可以使用摄像头推理
|
||
|
||
```shell
|
||
# 前往 mmdeploy 目录
|
||
cd ${PATH_TO_MMDEPLOY}/build/bin/
|
||
|
||
# 视频推理
|
||
./pose_tracker rtmpose-ort/rtmdet-nano/ rtmpose-ort/rtmpose-m/ your_video.mp4 --device cpu
|
||
|
||
required arguments:
|
||
det_model Detection 模型路径 [string]
|
||
pose_model Pose 模型路径 [string]
|
||
input 输入图片路径或摄像头序号 [string]
|
||
|
||
optional arguments:
|
||
--device 推理设备 "cpu", "cuda" [string = "cpu"]
|
||
--output 导出视频路径 [string = ""]
|
||
--output_size 输出视频帧的长边 [int32 = 0]
|
||
--flip 设置为1,用于水平翻转输入 [int32 = 0]
|
||
--show 使用`cv::imshow`时,传递给`cv::waitKey`的延迟;
|
||
-1: 关闭 [int32 = 1]
|
||
--skeleton 骨架数据的路径或预定义骨架的名称:
|
||
"coco", "coco-wholebody" [string = "coco"]
|
||
--background 导出视频背景颜色, "default": 原图, "black":
|
||
纯黑背景 [string = "default"]
|
||
--det_interval 检测间隔 [int32 = 1]
|
||
--det_label 用于姿势估计的检测标签 [int32 = 0]
|
||
(0 在 coco 中对应 person)
|
||
--det_thr 检测分数阈值 [double = 0.5]
|
||
--det_min_bbox_size 最小检测框大小 [double = -1]
|
||
--det_nms_thr NMS IOU阈值,用于合并检测到的bboxes和
|
||
追踪到的目标的 bboxes [double = 0.7]
|
||
--pose_max_num_bboxes 每一帧用于姿势估计的 bboxes 的最大数量
|
||
[int32 = -1]
|
||
--pose_kpt_thr 可见关键点的阈值 [double = 0.5]
|
||
--pose_min_keypoints 有效姿势的最小关键点数量,-1表示上限(n_kpts/2) [int32 = -1]
|
||
--pose_bbox_scale 将关键点扩展到 bbox 的比例 [double = 1.25]
|
||
--pose_min_bbox_size 最小追踪尺寸,尺寸小于阈值的 bbox 将被剔除 [double = -1]
|
||
--pose_nms_thr 用于抑制重叠姿势的 NMS OKS/IOU阈值。
|
||
当多个姿态估计重叠到同一目标时非常有用 [double = 0.5]
|
||
--track_iou_thr 追踪 IOU 阈值 [double = 0.4]
|
||
--track_max_missing 最大追踪容错 [int32 = 10]
|
||
```
|
||
|
||
**API** **示例**
|
||
|
||
\- [`pose_tracker.py`](https://github.com/open-mmlab/mmdeploy/blob/main/demo/python/pose_tracker.py)
|
||
|
||
\- [`pose_tracker.cxx`](https://github.com/open-mmlab/mmdeploy/blob/main/demo/csrc/cpp/pose_tracker.cxx)
|
||
|
||
## 📚 常用功能 [🔝](#-table-of-contents)
|
||
|
||
### 🚀 模型测速 [🔝](#-table-of-contents)
|
||
|
||
如果需要测试模型在部署框架下的推理速度,MMDeploy 提供了方便的 `tools/profiler.py` 脚本。
|
||
|
||
用户需要准备一个存放测试图片的文件夹`./test_images`,profiler 将随机从该目录下抽取图片用于模型测速。
|
||
|
||
```shell
|
||
python tools/profiler.py \
|
||
configs/mmpose/pose-detection_simcc_onnxruntime_dynamic.py \
|
||
{RTMPOSE_PROJECT}/rtmpose/body_2d_keypoint/rtmpose-m_8xb256-420e_coco-256x192.py \
|
||
../test_images \
|
||
--model {WORK_DIR}/end2end.onnx \
|
||
--shape 256x192 \
|
||
--device cpu \
|
||
--warmup 50 \
|
||
--num-iter 200
|
||
```
|
||
|
||
测试结果如下:
|
||
|
||
```shell
|
||
01/30 15:06:35 - mmengine - INFO - [onnxruntime]-70 times per count: 8.73 ms, 114.50 FPS
|
||
01/30 15:06:36 - mmengine - INFO - [onnxruntime]-90 times per count: 9.05 ms, 110.48 FPS
|
||
01/30 15:06:37 - mmengine - INFO - [onnxruntime]-110 times per count: 9.87 ms, 101.32 FPS
|
||
01/30 15:06:37 - mmengine - INFO - [onnxruntime]-130 times per count: 9.99 ms, 100.10 FPS
|
||
01/30 15:06:38 - mmengine - INFO - [onnxruntime]-150 times per count: 10.39 ms, 96.29 FPS
|
||
01/30 15:06:39 - mmengine - INFO - [onnxruntime]-170 times per count: 10.77 ms, 92.86 FPS
|
||
01/30 15:06:40 - mmengine - INFO - [onnxruntime]-190 times per count: 10.98 ms, 91.05 FPS
|
||
01/30 15:06:40 - mmengine - INFO - [onnxruntime]-210 times per count: 11.19 ms, 89.33 FPS
|
||
01/30 15:06:41 - mmengine - INFO - [onnxruntime]-230 times per count: 11.16 ms, 89.58 FPS
|
||
01/30 15:06:42 - mmengine - INFO - [onnxruntime]-250 times per count: 11.06 ms, 90.41 FPS
|
||
----- Settings:
|
||
+------------+---------+
|
||
| batch size | 1 |
|
||
| shape | 256x192 |
|
||
| iterations | 200 |
|
||
| warmup | 50 |
|
||
+------------+---------+
|
||
----- Results:
|
||
+--------+------------+---------+
|
||
| Stats | Latency/ms | FPS |
|
||
+--------+------------+---------+
|
||
| Mean | 11.060 | 90.412 |
|
||
| Median | 11.852 | 84.375 |
|
||
| Min | 7.812 | 128.007 |
|
||
| Max | 13.690 | 73.044 |
|
||
+--------+------------+---------+
|
||
```
|
||
|
||
如果你希望详细了解 profiler 的更多参数设置与功能,可以前往 [Profiler Docs](https://mmdeploy.readthedocs.io/en/main/02-how-to-run/useful_tools.html#profiler)
|
||
|
||
### 📊 精度验证 [🔝](#-table-of-contents)
|
||
|
||
如果需要测试模型在部署框架下的推理精度,MMDeploy 提供了方便的 `tools/test.py` 脚本。
|
||
|
||
```shell
|
||
python tools/test.py \
|
||
configs/mmpose/pose-detection_simcc_onnxruntime_dynamic.py \
|
||
{RTMPOSE_PROJECT}/rtmpose/body_2d_keypoint/rtmpose-m_8xb256-420e_coco-256x192.py \
|
||
--model {PATH_TO_MODEL}/rtmpose_m.pth \
|
||
--device cpu
|
||
```
|
||
|
||
详细内容请参考 [MMDeploys Docs](https://github.com/open-mmlab/mmdeploy/blob/main/docs/zh_cn/02-how-to-run/profile_model.md)
|
||
|
||
## 📜 引用 [🔝](#-table-of-contents)
|
||
|
||
如果您觉得 RTMPose 对您的研究工作有所帮助,请考虑引用它:
|
||
|
||
```bibtex
|
||
@misc{https://doi.org/10.48550/arxiv.2303.07399,
|
||
doi = {10.48550/ARXIV.2303.07399},
|
||
url = {https://arxiv.org/abs/2303.07399},
|
||
author = {Jiang, Tao and Lu, Peng and Zhang, Li and Ma, Ningsheng and Han, Rui and Lyu, Chengqi and Li, Yining and Chen, Kai},
|
||
keywords = {Computer Vision and Pattern Recognition (cs.CV), FOS: Computer and information sciences, FOS: Computer and information sciences},
|
||
title = {RTMPose: Real-Time Multi-Person Pose Estimation based on MMPose},
|
||
publisher = {arXiv},
|
||
year = {2023},
|
||
copyright = {Creative Commons Attribution 4.0 International}
|
||
}
|
||
|
||
@misc{mmpose2020,
|
||
title={OpenMMLab Pose Estimation Toolbox and Benchmark},
|
||
author={MMPose Contributors},
|
||
howpublished = {\url{https://github.com/open-mmlab/mmpose}},
|
||
year={2020}
|
||
}
|
||
```
|